Electron microscopy is the primary method for analyzing nanostructures. Senior researcher Thomas Willum Hansen discusses the new challenges in this field
Electron microscopy has for more than 50 years been the de facto tool for analyzing nanostructures such as nanoparticles. Over the years, the technique has progressed allowing spatial resolution at the sub-Ångstrøm level and energy resolution approaching a few tens of eV. More recently, focus has been on the speed and sensitivity the recording media used for acquiring the images in the microscope. This means that state of the art cameras are capable of recording images with frame rates exceeding 100 frames per second. The field now faces the challenge of analyzing vast amounts of data, naturally demanding automated approaches. Deep learning algorithms is a promising approach for detecting and analyzing the periodic arrangements of atoms observed in high resolution transmission electron micrographs.
Application of convolutional neural networks (CNN) is a viable route to analyze nanostructures such as the surface dynamics involved e.g. nanostructured graphene and the surfaces of catalytically active nanoparticles [1]. In both these cases, the exact position and dynamics of the surface atoms is a governing factor for the performance of the material. In order for the CNN to make substantiated predictions, it needs to be trained on images of the same kind as those to be analyzed. For this purpose, simulations can be used.
In order for the CNN to be able to analyze a structure, it needs to be trained on images similar to the experimental data which it is meant to analyze. However, it also needs to be able to correctly identify atomic positions, which are not necessarily in their ideal position. In the case of graphene, the model is created using a Voronoi tessellation of 2D data points mainly consisting of hexagons. From this structure, a hole is cut out to create surfaces. Using this structure, a set of image simulations are created by varying the image parameters of the microscope.
Figure 1 shows the performance of the CNN applied to an experimental image acquired in an aberration corrected FEI Titan transmission electron microscope operated at 80 kV. The low acceleration voltage was chosen as it is below the theoretical knock-on damage threshold in pristine graphene. The CNN accurately identifies the position of the atoms in the structure inside the graphene sheet. At the surface, the CNN outputs a probability for finding an atom at a given position.
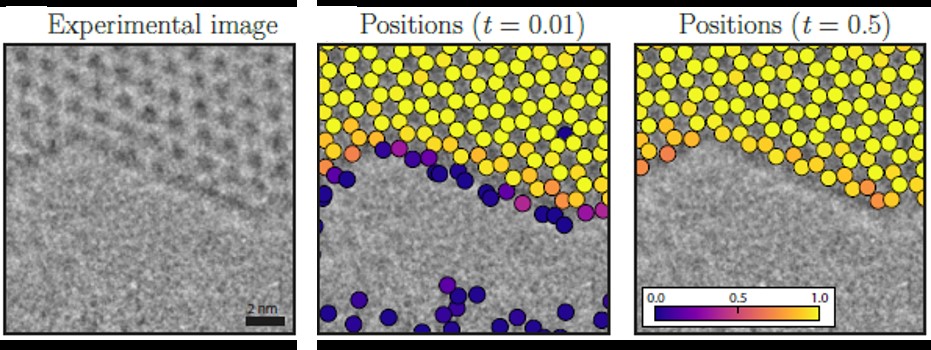
For analyzing images of gold nanoparticles, an active catalyst for e.g. the oxidation of toxic carbon monoxide to carbon dioxide, structures of gold of varying sizes and varying orientations are simulated. In order to increase the ability of the CNN to detect various structures, atoms are added to the models.
Experimental images of gold nanoparticles were obtained using an environmental FEI Titan transmission electron microscope where the samples can be exposed to low pressures of gas. The microscope was operated at 300 kV. An image sequence of a particle exposed to 4.5 Pa oxygen forms the experimental data.
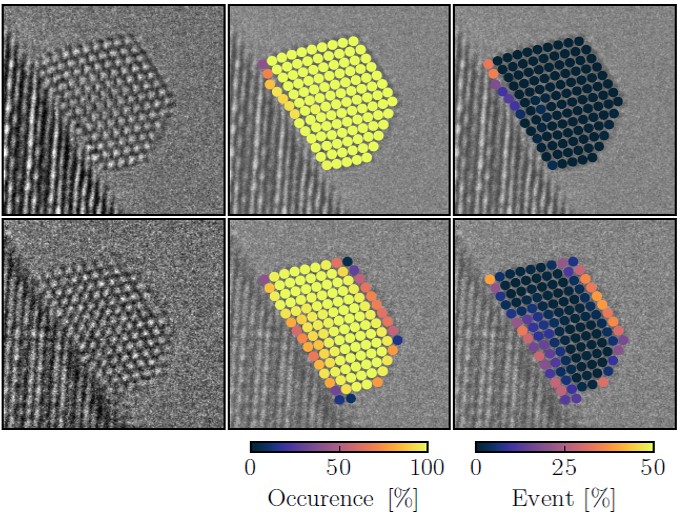
The analysis shows that he CNN accurately identifies the atomic columns in the bulk of the nanoparticle. At the surface of the particle, the atomic columns continuously shift around giving new surface configurations indicating that the surface of a catalyst is a highly dynamic entity, see Figure 2. The analysis also indicates that the lattice spacing changes going from the interior of the particle to the surface which also changes to electronic density at the surface, a factor which could govern the catalytic activity.
Deep learning in the form of convolutional neural networks is a viable approach to analyzing large datasets from transmission electron microscopy. When well-training, these systems can accurately analyze datasets much faster than a human being and with a much higher degree of objectivity.
Reference
[1]: Advanced Theory and Simulations 2018, 1800037 (2018), J. Madsen, P. Liu, J. Kling, J. B. Wagner, T. W. Hansen, O. Winther, J. Schiøtz, ‘A Deep Learning Approach to Identify Local Structures in Atomic-Resolution Transmission Electron Microscopy Images’
Senior researcher Thomas Willum Hansen
Center for Electron Nanoscopy
DTU Cen, Technical University of Denmark
Tel: +45 4525 6476