Utilising early cancer detection: are we getting the most out of first-order tests for symptomatic cancer patients?
At PinPoint Data Science we are committed to helping the NHS realise its ambitious target for the early detection of 75% of all cancers by 2028. Working with NHSEI, the National Cancer Team and Cancer Alliances we have been gathering ‘real-world evidence’ that is set to revolutionise cancer diagnostics in the UK and beyond. We aim to deploy our first products into clinical practice by the end of 2022. Here we explain the current scenario and how The PinPoint Test, an advanced ‘Multi-Cancer Early Detection’ test, will make a difference.
The way our healthcare system currently manages patients who seek help for worrying symptoms is based on the fundamental approach of taking a history, conducting an examination and usually the first order of diagnostic tests – predominantly blood tests. Thereafter, a differential diagnosis is typically worked through by a combination of consecutive further tests and referral for specialist advice, potentially from more than one Consultant. Eventually, a diagnosis is narrowed down but with a heavy reliance on costly and often invasive second-order testing. It can be a long and drawn-out process.
What if, at the very start of the patient pathway, the first-order test gave better, more insightful information? Could it shorten the differential diagnosis list? Could it more accurately determine the next step and most appropriate specialism? Could it prioritise the second-order test a patient requires and how urgently?
As a patient, what is the question you want to be answered when you receive a diagnostic test? Very simply, you want to know what (if anything) is wrong with you, so that your doctor can help you if needed or reassure you if not.
But is this the question current diagnostic tests answer? Well, sometimes.
The problem with complexity
Let’s consider blood tests. If you’re really lucky, there’s a single analyte the lab can measure that will give your doctor a clear answer. If not, there may be a combination of analytes that when looked at together, can provide an answer, but often this isn’t the case and the road to diagnosis becomes longer and less clear.
The human brain has evolved to recognise patterns and extrapolate conclusions, but there are limits to the number of interdependent data points we can process effectively at any one time. With a data set as complex and fluid as an individual patient’s biology, the absence of a red-flag test result can leave even the most experienced GP or Consultant forced to rely on intuition and a process of elimination and deduction.
But what if the answer is available in that first blood test, it’s just spread across tens or hundreds of analytes?
Cancer is a particularly challenging example. While there are a few specific cancers where a single analyte is useful (e.g., PSA for prostate cancer), for most cancers this is not the case. And yet, scientific literature reports many examples of signals that can be measured in blood and that are known to carry trace amounts of cancer information.
The difficulty is that these individual analytes are often not informative enough on their own to be useful in forming a diagnosis. An elevated platelet count might be a warning sign for cancer [1]; a range of immune-related biomarkers are associated with increased cancer risk [2]; C-reactive protein (CRP) can be elevated in ovarian cancer patients [3] and is even more significant when combined with CA-125 and HE4; serum bilirubin levels are negatively associated with both lung and cervical cancer risk [4]. The list goes on.
Some tumour markers already in use are more broadly informative than for just the cancers they are primarily used to investigate. For example, in primary care patients with elevated CA-125 (an ovarian cancer tumour marker), not only were 10.1% of patients diagnosed with ovarian cancer but a further 12.3% were diagnosed with a completely different type of cancer [5].
Despite the name, Prostate Specific Antigen (PSA) is known to also be produced at lower levels by other tissues and to have potential as a marker for colon and breast cancer [6, 7].
All of these analytes, and others not discussed here but linked to cancer risk, are easily measured in NHS pathology laboratories. They are routine, established and importantly, they are affordable to process. Without the right tool to interpret them, however, these analytes are a largely untapped resource.
A test with the ability to combine these individual signals into a single, more informative, calibrated result could be transformational for early diagnosis.
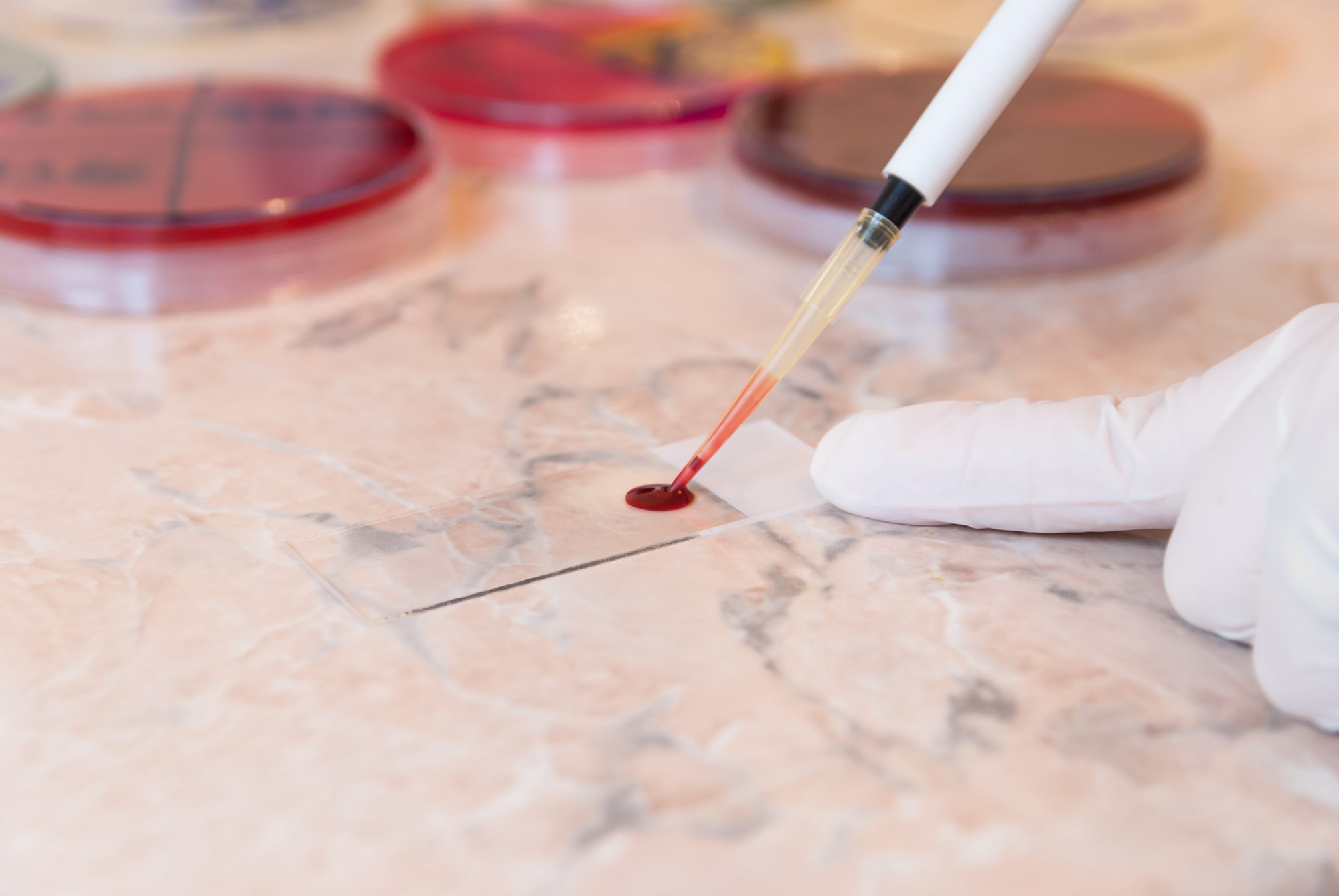
Machine learning: unlocking the next generation of blood tests
The PinPoint Test is a software in vitro diagnostic device for the detection of cancer across 9 referral pathways, representing 98% of all suspected cancer referrals in the NHS. The algorithm employs machine learning to analyse 31 standard analytes, plus the patient’s age and sex. It can calibrate and aggregate these individual signals into one strong and highly accurate result: the chance that a patient has cancer.
The result is designed to be a tool for supporting clinicians in the diagnostic process. It does what a person cannot: accurately calculate a patient’s risk profile for cancer-based on data from more patients than a GP could see in a lifetime. A simple answer to a complex question.
The PinPoint Test isn’t alone. There are several different approaches to multi-cancer early detection that are at various stages of development. Many of those more widely known is focused on the detection of circulating tumour DNA (ctDNA) in the blood. Companies in this area include GRAIL [8], CancerSEEK [9], Freenome [10] and Guardant [11], all of whom are developing tests that identify multiple pieces of information from ctDNA and in some cases, other analytes.
Just like PinPoint, tests such as that being developed by GRAIL also use machine learning to analyse data and produce a probability of cancer; and just like PinPoint, all of these tests are sensitive to multiple cancers. The potential for ctDNA testing in future screening programmes is exciting but PinPoint addresses a more immediate problem faced by the NHS today: systemic overloading in cancer services and the lack of an affordable tool to accurately stratify patients at, or potentially before, the start of an urgent cancer referral.
Unlike many of our counterparts, PinPoint does not require investment in new physical infrastructure. Blood samples are processed using standard equipment in NHS labs and the algorithm exists within NHS IT systems for data security and ease of use. As a result, it can be adopted at high-speed and low-cost across regions.
“An algorithm will never replace a clinician”
The trend is clear: to get the most out of first-order testing, we need to apply new tools to an old process. A test like PinPoint can cut through the complexity of medical data to produce an accurate and repeatable result. This new generation of tests should give doctors extra confidence in their decision-making process. An algorithm will never replace a clinician but like any other medical device, it will answer questions and perhaps raise valuable new ones.
We live in an era where artificial intelligence, machine learning algorithms, and statistical models are increasingly able to offer insight and constructive support in the clinical space. After Deep Blue defeated Gary Kasparov in the late 90s, the best chess players weren’t computers, they were teams of humans using computers to help them. The chess-playing software became a useful tool, and one that gave the human experts new perspectives to help them identify a more effective and successful route forwards. This is a useful way of thinking about the new generation of blood test-based clinical decision support systems for cancer. They provide new sources of information for the trained clinical expert; they can support and enhance a doctor’s clinical intuition; they are new tools with which to diagnose and guide treatment for patients.
Far from being a threat to that vital human element in healthcare, the next generation of blood tests will be a powerful ally for doctors and patients alike.
References
- Giannakeas,V., Kotsopoulos, J., Cheung MC. et al. Analysis of Platelet Count and New Cancer Diagnosis Over a 10-Year Period. JAMA Netw Open. 2022;5(1):e2141633. o https://jamanetwork.com/journals/jamanetworkopen/fullarticle/2787932
- Nøst TH, Alcala K, Urbarova I, Byrne KS, Guida F, Sandanger TM, Johansson M. Systemic inflammation markers and cancer incidence in the UK Biobank. Eur J Epidemiol. 2021 Aug;36(8):841-848. o https://pubmed.ncbi.nlm.nih.gov/34036468/
- Yang, D., Li, H., Sun, X. et al. Clinical usefulness of high levels of C-reactive protein for diagnosing epithelial ovarian cancer. Sci Rep 10, 20056 (2020). o https://www.nature.com/articles/s41598-020-77167-y
- Inoguchi, T., Nohara, Y., Nojiri, C. et al. Association of serum bilirubin levels with risk of cancer development and total death. Sci Rep 11, 13224 (2021). o https://www.nature.com/articles/s41598-021-92442-2
- Funston, G., Hamilton, W., Abel, G., Crosbie, E.J., Rous, B. and Walter, F.M., 2020. The diagnostic performance of CA125 for the detection of ovarian and non-ovarian cancer in primary care: A population-based cohort study. PLoS medicine, 17(10), p.e1003295. https://journals.plos.org/plosmedicine/article?id=10.1371/journal.pmed.1003295
- Pérez-Ibave DC, Burciaga-Flores CH, Elizondo-Riojas MÁ. Prostate-specific antigen (PSA) as a possible biomarker in non-prostatic cancer: A review. Cancer Epidemiology. 2018; 54:48-55 o https://www.sciencedirect.com/science/article/pii/S1877782118300936
- Black, M.H., Giai, M., Ponzone, R., Sismondi, P., Yu, H. and Diamandis, E.P., 2000. Serum total and free prostate-specific antigen for breast cancer diagnosis in women. Clinical cancer research, 6(2), pp.467-473. o https://aacrjournals.org/clincancerres/article/6/2/467/287977/Serum-Total-and-Free-Prostate-specific-Antigen-for
- Klein, E.A., Richards, D., Cohn, A., Tummala, M., Lapham, R., Cosgrove, D., Chung, G., Clement, J., Gao, J., Hunkapiller, N. and Jamshidi, A., 2021. Clinical validation of a targeted methylation-based multi-cancer early detection test using an independent validation set. Annals of Oncology, 32(9), pp.1167-1177. o https://www.sciencedirect.com/science/article/pii/S0923753421020469
- Cohen, J.D., Li, L., Wang, Y., Thoburn, C., Afsari, B., Danilova, L., Douville, C., Javed, A.A., Wong, F., Mattox, A. and Hruban, R.H., 2018. Detection and localization of surgically resectable cancers with a multi-analyte blood test. Science, 359(6378), pp.926-930. https://www.science.org/doi/abs/10.1126/science.aar3247
- Wan, N., Weinberg, D., Liu, T.Y., Niehaus, K., Ariazi, E.A., Delubac, D., Kannan, A., White, B., Bailey, M., Bertin, M. and Boley, N., 2019. Machine learning enables detection of early-stage colorectal cancer by whole-genome sequencing of plasma cell-free DNA. BMC cancer, 19(1), pp.1-10. https://bmccancer.biomedcentral.com/articles/10.1186/s12885-019-6003-8
- Lee, J., Kim, HC., Kim, ST. et al. Multimodal circulating tumour DNA (ctDNA) colorectal neoplasia detection assay for asymptomatic and early-stage colorectal cancer (CRC). https://ascopubs.org/doi/abs/10.1200/JCO.2021.39.15_suppl.3536
Please note: This is a commercial profile
© 2019. This work is licensed under CC-BY-NC-ND.