A unique Artificial Intelligence-based deep learning model can identify birth defects like cystic hygroma in fetal ultrasounds early on
Researchers are testing unique AI as an assistive tool for the rapid and accurate reading of ultrasound images which could have major implications for mothers and babies around the globe. It could be the beginning of a revolution in AI identification of birth abnormalities, the lead researcher believes.
Cystic hygroma is a rare and potentially life-threatening disorder. The embryonic condition causes fluid to swell around the head and neck so that the lymphatic vascular system develops abnormally.
The new proof-of-concept study, led by Dr. Mark Walker at the University of Ottawa’s Faculty of Medicine, aims to demonstrate the potential for deep-learning architecture to support early and reliable identification of cystic hygroma from first trimester ultrasound scans.
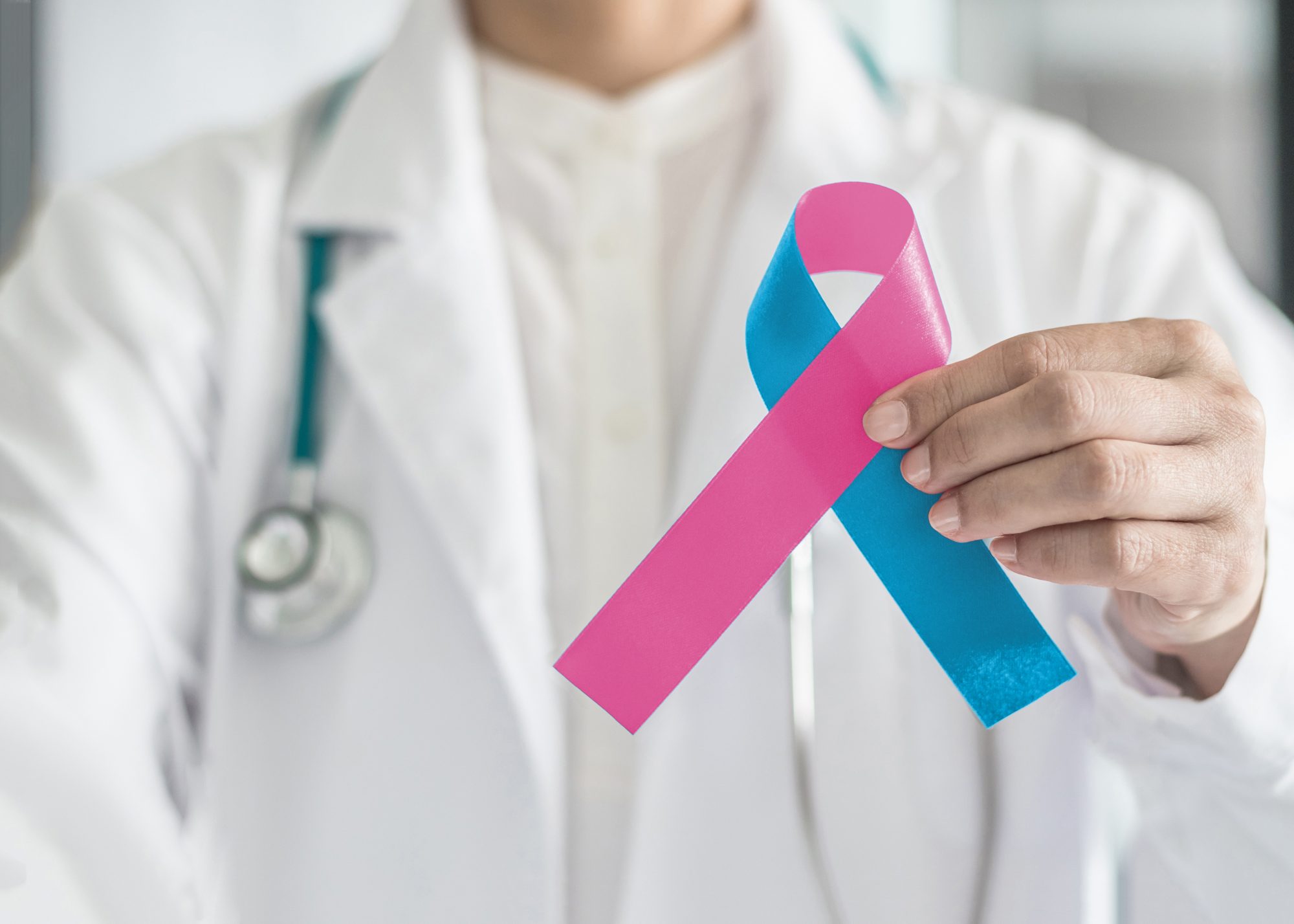
Revolutionising approaches to identifying cystic hygroma
Cystic hygroma, sometimes referred to as a lymphatic malformation, cannot be prevented because it occurs so early in the pregnancy – normally before the pregnancy is confirmed.
It is not clear why lymphatic malformations occur. Nothing done or not done during pregnancy causes the cystic hygroma to develop. They occur in approximately 1% of children regardless of sex and gender.
Therefore, if AI can detect this kind of birth abnormality then it really should be considered revolutionary because currently, this type of technology does not exist.
Although the birth defect can typically be easily diagnosed prenatally during an ultrasound appointment, Dr. Walker, co-founder of the OMNI Research Group, and his research team wanted to prove that AI-driven pattern recognition could also do the job.
“What we demonstrated was in the field of ultrasound we’re able to use the same tools for image classification and identification with a high sensitivity and specificity,” says Dr. Walker.
This discovery may mean that other fetal anomalies, normally identified by ultrasonography, can be diagnosed with AI instead.