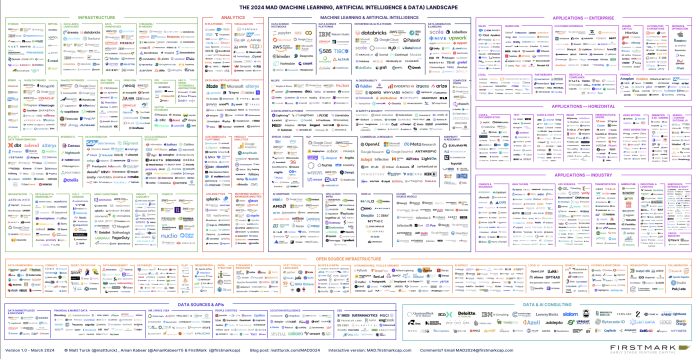
Drawing on real-world experience and research involving over 4,000 AI use cases, Nortal provides insights into how governments can best utilise AI
At Nortal, we have analysed 4,372 AI use cases, including 750 publicly available cases across 30 countries, to understand what works and what doesn’t. Our conclusion: Governments don’t need more AI; they need the right AI – built for the realities of public service, integrated into existing workflows, and implemented with frontline professionals, not imposed on them. When AI fails in government, it’s rarely because the technology isn’t good enough. It’s because it wasn’t built for the job.
We drew three main conclusions about AI use cases:
1. Start with a reality check: know what’s possible in a given sector
How: AI should be deployed where it directly enhances existing systems, avoiding disruption. The key is understanding the sector’s needs, current operations, and existing solutions before introducing new technology.
Example: In Sydney, the road maintenance department solved the challenge of pothole detection by adapting existing infrastructure (garbage trucks) rather than introducing disruptive technology. By mounting smartphones on these trucks, which covered most roads, they created a real-time scanning system that detected potholes. This approach leveraged existing workflows without requiring significant infrastructure changes or additional vehicles, maximising efficiency.
2. You can’t make government fit AI, so you have to make AI fit government
- How:
- AI must be integrated into government processes to address specific, targeted challenges. Governments should focus on gradual, manageable adoption that targets high-impact use cases without disrupting core public services.
- Example:
- Estonia’s AI Leap 2025 program exemplifies this approach by offering teachers and students AI tools like OpenAI’s ChatGPT. This is not a blanket AI push; the programme starts with a select number of students and will expand based on feedback. Teacher training is a core component to ensure the effective integration of AI into existing educational methods, demonstrating how AI can be introduced in a controlled and impactful way.
3. Proper digital foundations ensure AI doesn’t remain a pilot project
- How:
- For AI to scale and have a meaningful impact, it must be supported by a strong digital infrastructure. This means ensuring that data is interoperable, high-quality, and secure and that governance is in place to manage it. Without these foundational elements, AI can only function in isolated pilot projects, not as a core capability across the government.
- Example:
- Singapore’s Smart Nation initiative demonstrates this approach. The government integrated AI within a broader digital strategy, ensuring AI tools had access to well-structured, secure data across public agencies. This created a unified data backbone, enabling AI applications to scale efficiently.
Case study: tackling unemployment with AI
The Estonian Unemployment Investment Fund (EUIF) faced a massive challenge during the COVID-19 crisis. With just 350 counsellors, the fund had to handle 96,000 unemployment cases. Each counsellor was responsible for 274 cases, overwhelming the system.
To resolve this, Nortal worked with the Center for IT Impact Studies to create an AI-driven decision-support tool that analysed each case, predicted reemployment probabilities, and flagged high-risk individuals who needed additional support. This allowed counsellors to make data-driven, personalised decisions at scale, improving efficiency and outcomes.
This success story highlights Estonia’s advantage: a public sector ready for AI. Many governments struggle with messy, siloed data that can undermine even the most advanced AI initiatives. Without structured, high-quality, accessible data, AI can mislead and underperform.
Four essential questions
There are plenty of examples of AI-enabling government initiatives if you know where to look. The UK’s National AI Strategy, Canada’s Algorithmic Impact Assessment, Finland’s national AI training program, and Estonia’s X-Road, to name a few. What do they likely have in common? Strong and accountable leadership that asked the right questions from the outset.
1. What are your expectations of ROI?
Have you defined the problem clearly? Is AI the right solution, or might another solution be better? Focusing on ROI helps you answer these questions, ensuring the AI solution addresses the real problem. This will justify the costs and resources required, with clear KPIs and a way to measure success.
2. What are your budget constraints?
Don’t forget to factor in long-term costs for maintenance, updates, and the experts you’ll need for deployment and ongoing support. Consider testing iteratively to lower the risk of poor outcomes and deploy in a select area before scaling up.
3. What are the data challenges and legal restrictions?
AI thrives on data, so ensure you understand the required data, availability, and quality. Be aware of data privacy and security concerns and ensure compliance with regulations.
4. How savvy are your citizens?
Assess how much training and support users will need to adopt AI solutions. Public opinion and ethical implications are critical for adoption. Does your solution ensure fairness, transparency, and accountability? How will it integrate with existing workflows and processes?
Ask us your questions
From streamlining public services in Germany to developing world-leading digital systems in Estonia, Nortal has proven its ability to transform complex government operations into world-leading digital systems. In the UK, we are focused on simplifying services and building the digital backbone for integrated, citizen-first solutions. With our experience in delivering successful e-government solutions aligned with the UK’s digital transformation agenda, we’re prepared to meet the evolving expectations of citizens and public sector needs.