Eric Buckland, PhD of Translational Imaging Innovations, delves into how we can achieve better transparency, traceability, and reproducibility in AI/ML for ophthalmology and medicine
Biomarkers are indispensable to finding cures and developing new therapies for degenerative eye diseases. Biomarkers are objectively measurable characteristics or indicators of normal biological processes, disease processes, or responses to therapeutic interventions. Biomarkers are essential to decision-making throughout drug development and patient care management.
Biomarkers in ophthalmology
In ophthalmology, biomarkers derived from bodily fluids, tissues, functional vision tests, and imaging are under constant development. New drug development relies on biomarkers to promote, redirect, or terminate therapeutic candidates at all phases of the drug development process.
More effective biomarkers are essential to increasing drug safety and effectiveness, reducing total development costs, and accelerating time to market. In the early phases of drug development, biomarkers play a critical role in categorizing pharmacodynamics.
At later stages, biomarkers help to define clinical outcomes. Biomarkers directly correlated to a patient’s journey through illness, including the progression of symptoms, changes in quality of life, and even survival, form an essential class of surrogate endpoints for clinical trials.
Biomarkers and clinical endpoints in ophthalmology
In ophthalmology, biomarkers and clinical endpoints is an area of active research to support innovations in treating macular degeneration, glaucoma, and inherited retinal diseases responsible for vision impairments impacting 200 million people worldwide.
Advancing new biomarkers and clinical endpoints necessitates using artificial intelligence (AI) in ophthalmology.
The techniques of AI are proving indispensable for completing complex data analysis tasks and uncovering relationships in images and data that may have been simply impossible without AI.
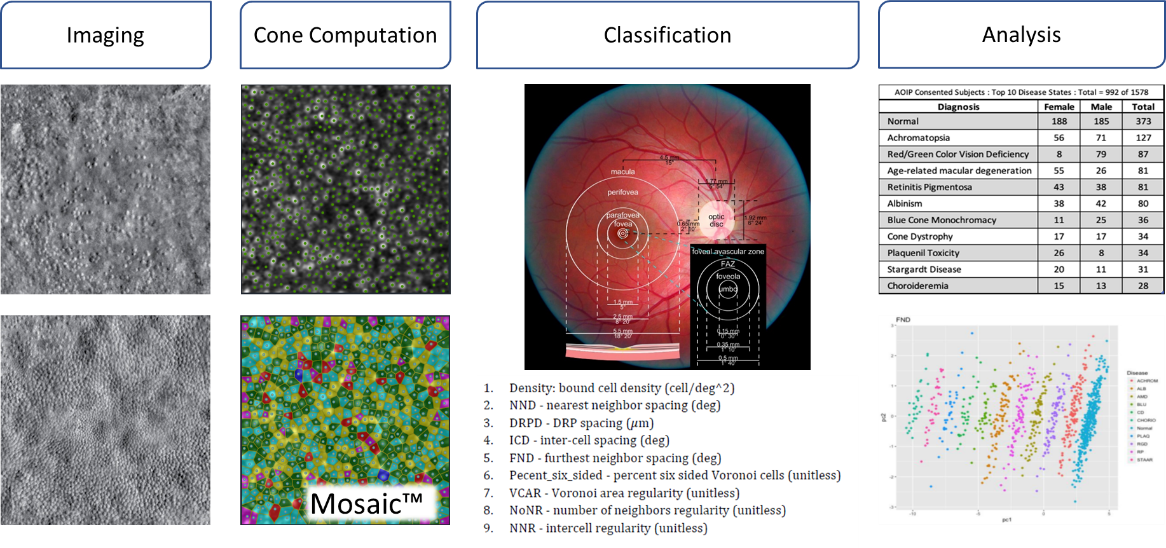
AI and ML for healthcare applications
A recent publication (1) provides a meta-analysis of activity in the Medical AI space: 130,000 papers were published, and $12 billion of venture capital was invested in 2021. For this investment, as of October 2022, the FDA lists 521 AI and Machine Learning (ML) Enabled Medical Devices (2); seven are in ophthalmology, and five clearances address one disease: Diabetic Retinopathy.
Developing AI for healthcare applications presents a different challenge than developing AI for consumer or business applications. For one, the stakes for patient healthcare outcomes are high. For another, access to training data is significantly more difficult. OpenAI deployed thousands of technicians scouring and annotating undisclosed scores of public domain records at the cost of hundreds of millions of dollars to develop the remarkable ChatGPT natural language processing (NLP) engine.
Medical AI requires both NLP and image processing, and the data – where digitally available – is protected in electronic health records and medical PACS systems. Data accessibility, problem complexity, and personal protections are among the challenges facing medical applications of AI/ML.
Regulatory and ethical considerations demand transparency, traceability, and reproducibility in AI/ML applied to biomarker development and clinical endpoint validation. If you have not had a chance to experiment with ChatGPT, I recommend you do so. Here is an abridged answer to a question that I recently posed (3):
How does biomarker development rely on transparency, traceability & reproducibility?
Biomarker development relies on transparency, traceability, and reproducibility to ensure the validity and reliability of the biomarker’s performance and its potential applications:
- Transparency: Transparency refers to the availability of clear and comprehensive information about the biomarker development process, including data sources, methodologies, and analytical techniques used. This transparency allows for critical assessment and facilitates the identification of potential biases, limitations, or sources of error.
- Traceability: Traceability involves maintaining a detailed record of all steps and decisions during the biomarker development process. Traceability allows for identifying and resolving any issues that may arise during development and the ability to reproduce the process or make improvements based on previous work.
- Reproducibility: Reproducibility is obtaining consistent and comparable results when repeating a study or experiment using the same methodology, data, and conditions. In biomarker development, reproducibility is crucial to validate the biomarker’s performance across different laboratories, populations, or clinical settings.
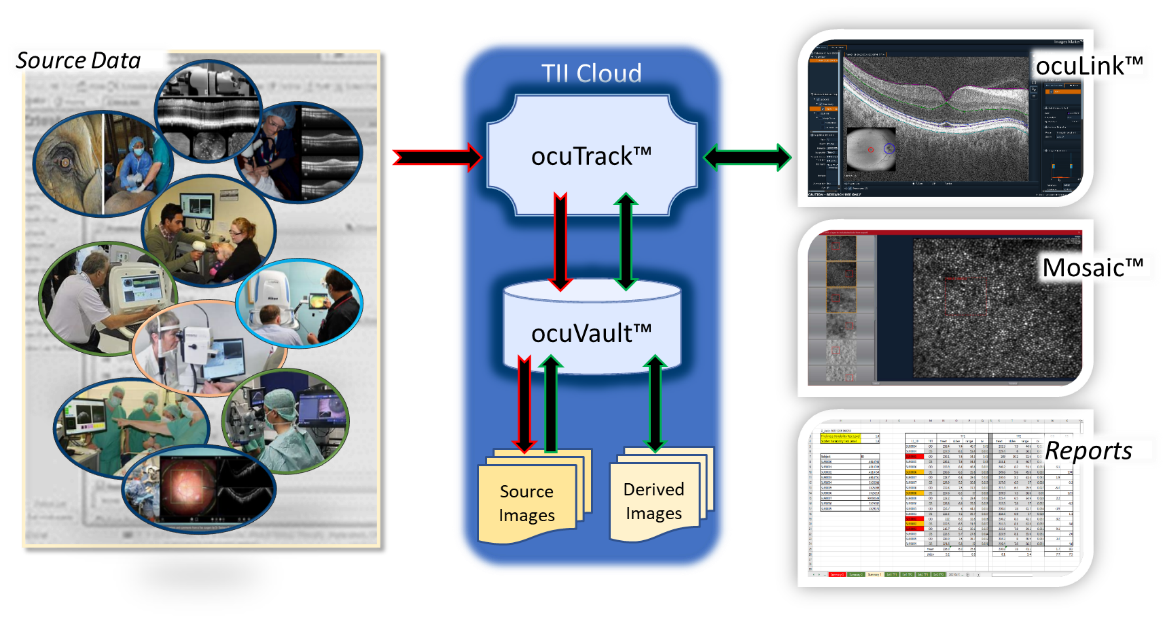
Clinicians, healthcare providers, and industry stakeholders rely on robust biomarkers that have been developed with rigorous methodologies and can be reproduced across different patient populations or settings. Transparency, traceability, and reproducibility in ophthalmology support the adoption and integration of biomarkers into clinical practice, ultimately benefiting patients and improving healthcare outcomes.
Accelerate biomarkers and clinical endpoints
Translational Imaging Innovations aims to accelerate the development and deployment of biomarkers and clinical endpoints and reduce the friction in AI/ML processes for medical applications. At the core of the Translational Imaging Innovations software suite are ocuVaultTM and ocuLinkTM.
ocuVaultTM is our advanced database constructed around the large volumes of images, data, and workflows that drive ophthalmic AI/ML development, biomarker discovery, clinical research, and clinical trials. ocuVaultTM provides registry, security, and accessibility of images and associated data to a growing library of application.
MosaicTM, referenced in the figures, is our computational engine for efficient batch processing of images for biomarker development and clinical research.
Whether locally deployed or deployed in the TII CloudTM, ocuVaultTM supports automated validations, analysis, and transfers of images and data in natural history studies and clinical trials. ocuVault-in-the-CloudTM (now called ocuTrackTM) provides convenience, traceability, and control in multi-site research projects and clinical trials.
ocuLinkTM is our flagship application for curating, visualizing, and annotating images. ocuLinkTM allows investigators to organize images into meaningful collections and grade, label, and annotate images for supervised or unsupervised algorithm development projects. ocuLinkTM is the perfect environment for automating multi-step workflows from biomarker discovery through automated analysis.
ocuLinkTM and ocuVaultTM provide the Transparency, Traceability, and Reproducibility required for the successful development and deployment of AI-enabled biomarkers and clinical endpoints:
- Build stable collections of images and data.
- Construct reusable and sharable palettes of labels and annotations.
- Link annotated collections to external AI/ML development environments.
- Label images, and train, test, and validate algorithms in managed projects linked to collections.
- De-identify and mask images and data for unbiased analysis.
- Trace all images, algorithms, and results with automated database registrations tied to unique identifier codes for all objects and processes.
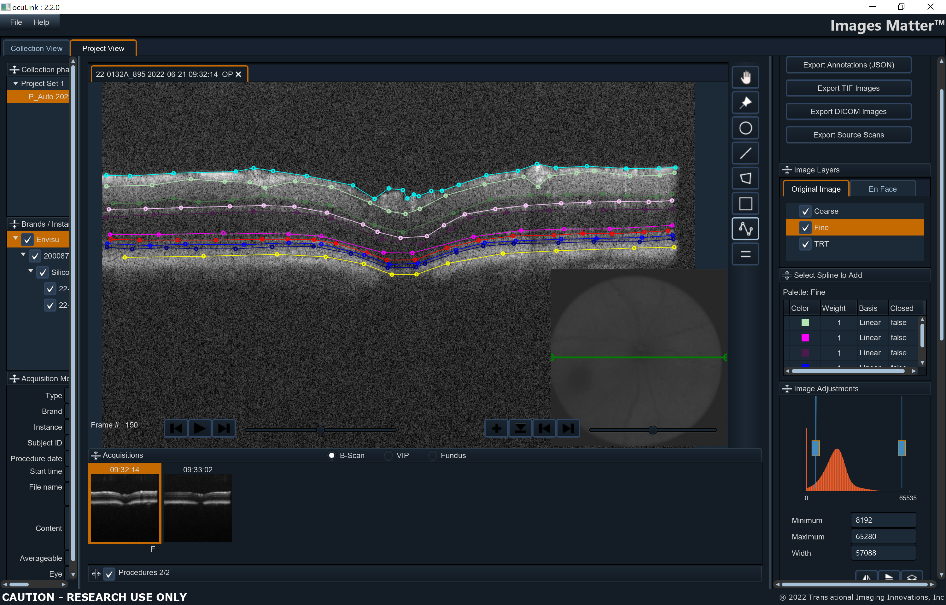
“Delivering the most convenient and productive solutions for ophthalmic biomarker discovery and clinical endpoint validation” (4) is the commitment of Translational Imaging Innovations. TII aims to enable translational researchers to develop better diagnostics and better therapies with more predictable benefits – faster, cheaper, and less frustrating.
References
- Karpov OE, et al. Analysis of Publication Activity and Research Trends in the Field of AI Medical Applications: Network Approach. International Journal of Environmental Research and Public Health. 2023; 20(7):5335. https://doi.org/10.3390/ijerph20075335
- https://www.fda.gov/medical-devices/software-medical-device-samd/artificial-intelligence-and-machine-learning-aiml-enabled-medical-devices?#resources
- OpenAI. (2023). How does biomarker development rely on transparency, traceability, and reproducibility? [ChatGPT]. In OpenAI. Retrieved June 5, 2023, from https://www.openai.com/
- https://hospinov.com/degenerative-eye-disease-and-the-opportunities-of/
Further reading
- https://www.openaccessgovernment.org/visual-impairment-eye-health-disease-biomarker-research/131871/