Dr. David S. Ebert from the University of Oklahoma’s Data Institute for Societal Challenges and Dr. Aaron Wendelboe from the University of Oklahoma Health Sciences Center outline how a cohesive, multidisciplinary, and multi-tiered approach can support a more predictive model in disease surveillance and control
Early detection of disease transmission has been a public health goal for decades. Syndromic and sentinel disease surveillance systems are two approaches designed to enhance early detection surpassing traditional, passive approaches.1 Public health disease surveillance is being conducted in countless settings, including healthcare, vertebrate and invertebrate animals, wastewater, air quality, transportation, and commercial activities.
Threats from pandemic influenza, bioterrorism, SARS1/MERS, antibiotic-resistant bacteria, and increased distribution of pathogens and insects, which threaten the environment, have been priority concerns for public health agencies.
Unfortunately, attaining the goal of early disease detection has been somewhat elusive. The current challenges include identifying optimal data sources, maintaining the ability to efficiently conduct ongoing disease surveillance that relies on a large and complex data network, and effectively analysing and summarising the data in a way that cuts through the ‘noise’ and identifies true events of public health concern.
Many other approaches to mitigate the impacts of current and potential health threats have been ongoing, such as the World Health Organization’s Health Emergencies Programme,2 the UK’s Influenza Pandemic Preparedness Strategy,3 and the USA’s National Pandemic Strategy.4 In addition, there is a history of including academic partners, such as the Centers for Disease Control and Prevention’s (CDC) Centers for Public Health Preparedness,5 as an innovative way to protect against severe pandemics.
Adopting a One Health approach to disease surveillance and control
Emphasis on taking a One Health approach to disease surveillance and control has been growing. The One Health approach capitalises on the interconnected human, animal, and environmental systems and emphasises coordinating, communicating, and collaborating among stakeholders who protect human, animal, and environmental health.6 While progress has been made since the First International One Health Congress was held in 2011,7 calls for improved application of One Health principles continued to be made early in the COVID-19 pandemic.8
Another key shortcoming of public health preparedness efforts is the insufficient collaboration between multidisciplinary experts, such as data scientists, computer engineers, anthropologists, social scientists, and systems engineers. Even collaborations within health-related fields, such as veterinary and environmental health, have been relatively weak.
Despite efforts, the SARS-CoV-2 virus still managed to cause a major pandemic unseen for a century. Furthermore, given that SARS-CoV-2 was first detected in a symptomatic patient9 (as opposed to earlier in the chain of disease emergence, such as in an animal host), it is evident improvements in disease surveillance methodology are required for earlier detection of pathogens of pandemic potential.
Multi-tiered approach to disease surveillance and control
To address these gaps in knowledge and preparedness, we are responding in a multi-tiered approach with a One Health perspective that will be economically feasible and sustainable, as summarised in Figure 1 (below).
To achieve this goal, we have identified and engaged a broad set of stakeholders, created broad multidisciplinary teams, are combining relevant data sources in innovative ways that will serve as early indicators, are using advanced technologies for early diagnosis, and advancing analytic methods to maintain high specificity for true event identification, while reducing the number of false positive alerts. Developing and implementing these approaches will shift disease surveillance and control for emerging infectious diseases from a solely reactive model to a more predictive model functioning in real time. The following section elaborates on our strategies and progress for each goal.
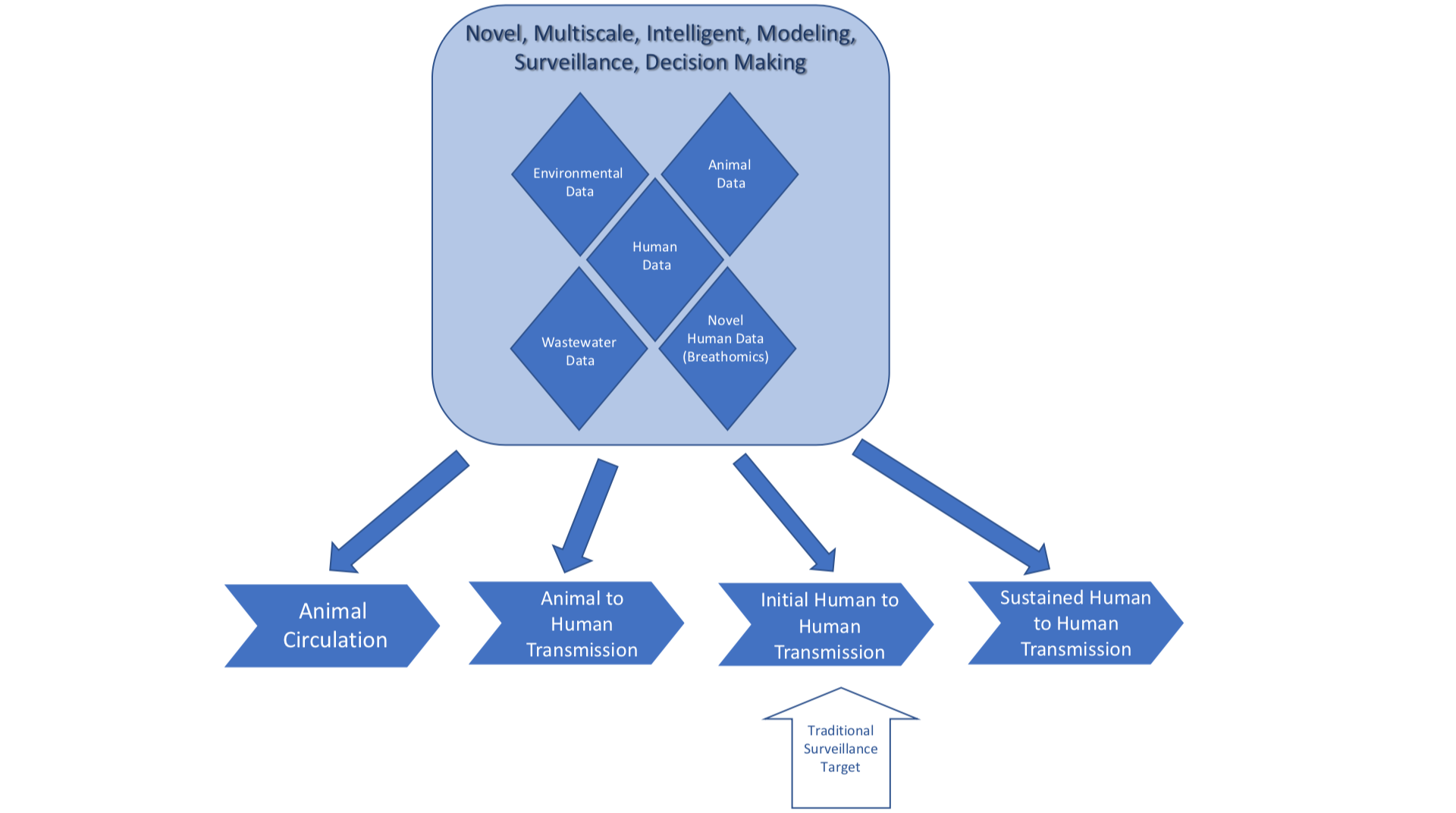
We met with government agencies and academic teams to engage stakeholders and described our project’s goals.
In this process, we have identified organisations and teams in Oklahoma and neighbouring states with complementary goals. We are working towards building and strengthening new collaborations to combine resources. In addition, we are holding regular webinars on topics covered by each team. Again, this has led to identifying new stakeholders who want to contribute to the success of our project.
We brought together experts in public health, medicine, veterinary science, data science, computer science, anthropology, informatics, and systems engineering to bring the needed skills to address the problem.
The data sources we are accessing include governmental disease surveillance, syndromic surveillance data from environmental data, ESSENCE/Biosense,10 wastewater surveillance data,11 veterinary data,12 and medical record data from the health information exchange.13 We will access environmental monitoring data from Earth-observing satellites and ground-based sensor networks.
The wastewater and river shed surveillance data are a key data source that intersects environmental, animal, and human data. In the past, these data sources have essentially acted as silos; we are combining them to help verify signals.
One exciting new technology we are using is in the field of breathomics research. We have developed a tool in which an individual’s breath can be analysed, and a diagnosis of COVID-19 can be made within one minute. The technology works by identifying specific volatile organic compound signatures from pathogens. We are in the process of extending this technology to be used to identify influenza and RSV.
The advanced analytic methods implement a data-driven syndromic and epidemiological model that harnesses novel AI-enabled methods to monitor, integrate, and correlate sources across various scales for pandemic detection and prediction. We leverage human-guided, predictive, information and interpretable AI/ML within methods for disease surveillance deployment, sampling, and analysis to manage these large data streams. Not only are we detecting alerts, but developing models to project the impact of an event if it goes undetected. These models are expected to help prioritise which signals must be investigated first.
By successfully achieving these five activities, we will be positioned to have an intelligent, interactive response planning and monitoring system that scales these new data sources and analytical methods across the community, state, and national levels and accounts for diverse population dynamics and disease spread, enabling earlier detection and response.
Corresponding author: Dr. Aaron Wendelboe, Department of Biostatistics and Epidemiology, Hudson College of Public Health, University of Oklahoma Health Sciences Center
References
- Meckawy R, Stuckler D, Mehta A, Al-Ahdal T, Doebbeling BN. Effectiveness of early warning systems in the detection of infectious diseases outbreaks: a systematic review. BMC Public Health. Nov 29 2022;22(1):2216. doi:10.1186/s12889-022-14625-4
- World Health Organization. WHO Health Emergencies Programme: Preparing for Pandemics. World Health Orgnaization. Accessed May 14, 2023. https://www.who.int/westernpacific/activities/preparing-for-pandemics
- DH Pandemic Influenza Preparedness Team. UK Influenza Pandemic Preparedness Strategy 2011. 2011. https://assets.publishing.service.gov.uk/government/uploads/system/uploads/attachment_data/file/213717/dh_131040.pdf
- Centers for Disease Control and Prevention. National Pandemic Strategy. Accessed May 14, 2023. https://www.cdc.gov/flu/pandemic-resources/national-strategy/index.html
- New academic centers for Public Health Preparedness funded. Public Health Rep. Nov-Dec 2002;117(6):592.
- Centers for Disease Control and Prevention. One Health Basics. Accessed May 14, 2023. https://www.cdc.gov/onehealth/basics/index.html
- Centers for Disease Control and Prevention. History. Accessed May 14, 2023. https://www.cdc.gov/onehealth/basics/history/index.html
- Ruckert A, Zinszer K, Zarowsky C, Labonte R, Carabin H. What role for One Health in the COVID-19 pandemic? Can J Public Health. Oct 2020;111(5):641-644. doi:10.17269/s41997-020-00409-z
- Steensma DP, Kyle RA. Dr Li Wenliang: Wuhan “Whistleblower” and Early COVID-19 Victim. Mayo Clin Proc. Jul 2022;97(7):1409-1410. doi:10.1016/j.mayocp.2022.05.033
- Gould DW, Walker D, Yoon PW. The Evolution of BioSense: Lessons Learned and Future Directions. Public Health Rep. Jul/Aug 2017;132(1_suppl):7S-11S. doi:10.1177/0033354917706954
- Kuhn KG, Jarshaw J, Jeffries E, et al. Predicting COVID-19 cases in diverse population groups using SARS-CoV-2 wastewater monitoring across Oklahoma City. Sci Total Environ. Mar 15 2022;812:151431. doi:10.1016/j.scitotenv.2021.151431
- Glickman LT, Moore GE, Glickman NW, Caldanaro RJ, Aucoin D, Lewis HB. Purdue University-Banfield National Companion Animal Surveillance Program for emerging and zoonotic diseases. Vector Borne Zoonotic Dis. Spring 2006;6(1):14-23. doi:10.1089/vbz.2006.6.14
- Tallman EF, Richardson D, Kendrick D, Dixon BE. Leveraging HIE to facilitate large-scale data analytics. Health Information Exchange. Academic Press; 2023:399-421.

This work is licensed under Creative Commons Attribution-NonCommercial-NoDerivatives 4.0 International.