In this paper, Professor Dane Morgan and Research Scientist Ryan Jacobs, from the University of Wisconsin, Madison, discuss their adventures in the field of machine learning in the areas of materials science and engineering
This paper gives a very brief and inevitably biased overview of machine learning (ML) in Materials Science and Engineering (MS&E), with examples taken from our own work with collaborators. We hope it conveys our excitement about the extraordinary potential of this new area of research.
MS&E focuses on developing materials with desired properties. It has led to materials innovations that underlie much of modern society, from the transistors in computers to the batteries in cars and smartphones. In recent decades, major advances in algorithms, computing power, and data access have made ML tools extremely powerful.
Our group and many others are exploring how ML tools can be used to enable new MS&E research and accelerate the process of materials discovery and optimization. (1)
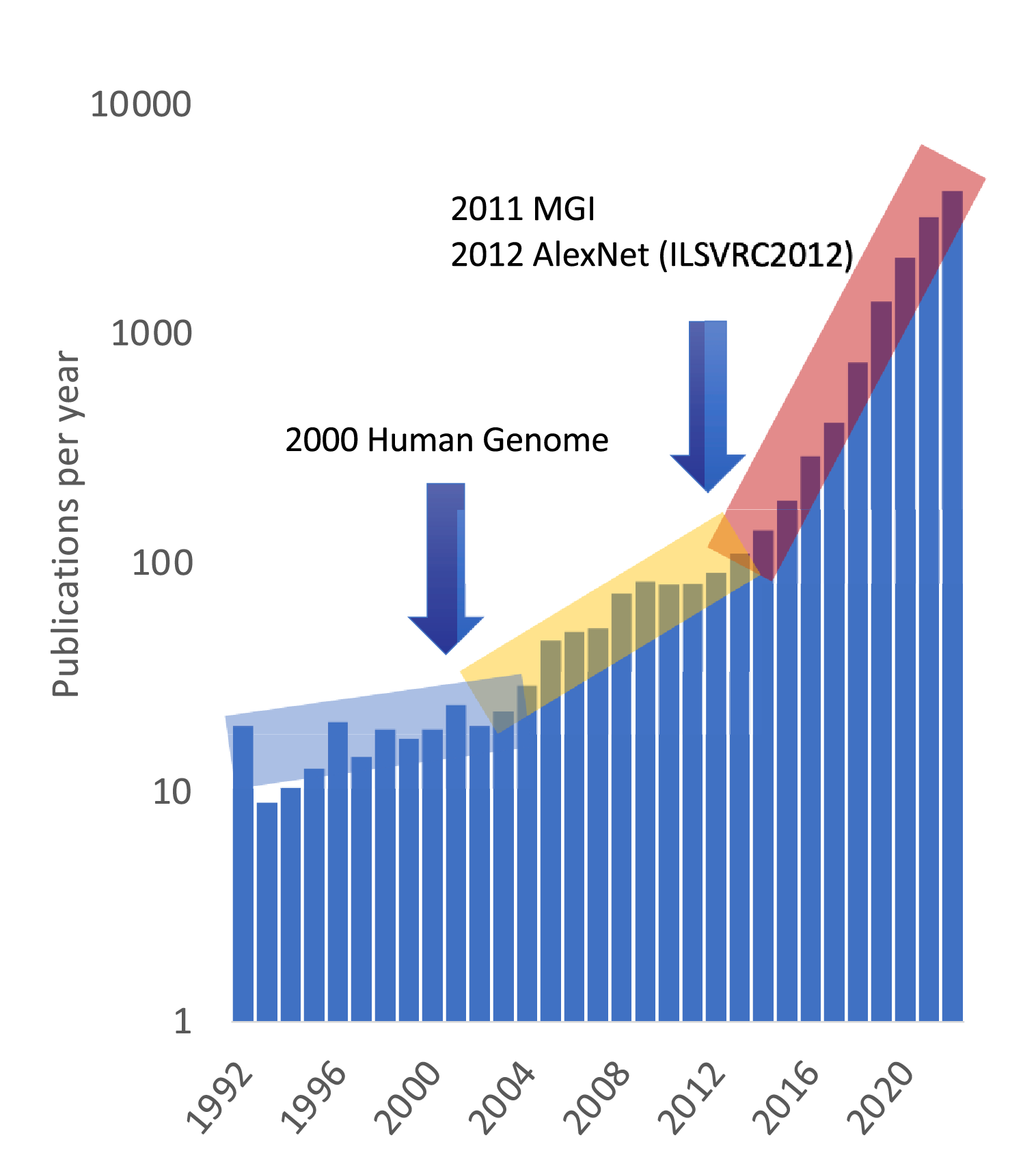
Figure 1 shows the approximate publication rate of papers on ML and materials science and engineering.
Two significant inflections can be seen in the data, which we speculate may relate to the popularization of data- centric thinking and funding opportunities that arose around the successful sequencing of the human genome in 2000, and then the nearly simultaneous adoption of the Materials Genome Initiative (MGI) in 2011 and the breakout success of deep learning in the ImageNet Large Scale Visual Recognition Challenge in 2012 (ILSVRC2012).
The role of ML in materials science and engineering
It is helpful to organize the role of ML in MS&E around three broad categories (i) property predictions, (ii) data-driven models, and (iii) machine interpretations.
Category (i) uses patterns in existing materials data to make property predictions on new materials, e.g., to guide future experiments or increase database sizes.
As an example in this category, we have worked with collaborators to develop models that predict radiation embrittlement of nuclear steels from their composition and irradiation conditions (2) [Figure 2(a)]. Our MAterials Simulation Toolkit – Machine Learning (MAST-ML) largely automates developing a wide range of such ML models, including uncertainty quantification, and is accessible for even novice users. (3)
Category (ii) refers to using data-driven ML models to reduce the needs for, or even replace, physics-based models. An outstanding example in this category are ML interatomic potentials (MLIPs), which can be trained to reproduce the key physics of atomistic processes on length and time scales thousands to millions of times larger than traditional fully quantum approaches. MLIPs are expected to enable many transformative applications, from designing better drugs to improving batteries. We have worked with collaborators to use MLIPs to model difficult-to-measure properties of molten salts useful for next-generation nuclear reactors (4) [Figure 2(b)].
Category (iii) refers to using ML to characterize and analyze data (typically in the form of images or text) of relevance for materials science and engineering, generally attempting to replace tasks that are inefficient or impossible for humans to do well. As an example of this category, we have worked with collaborators to develop deep learning machine vision models that can automatically find radiation damage defects in electron microscope images of irradiated metals (5) [Figure 2(c)], allowing orders of magnitude more defects to be studied than previous human-based approaches.
We expect these tools to provide a qualitative change in our ability to understand radiation effects and eventually help design materials for safer and cheaper nuclear fission and fusion reactors. Another example is our work demonstrating that simple screening and careful prompt engineering can allow large language models to extract materials property data from millions of papers with almost no human effort (6), opening the door to unprecedented levels of rapid data extraction and analysis.
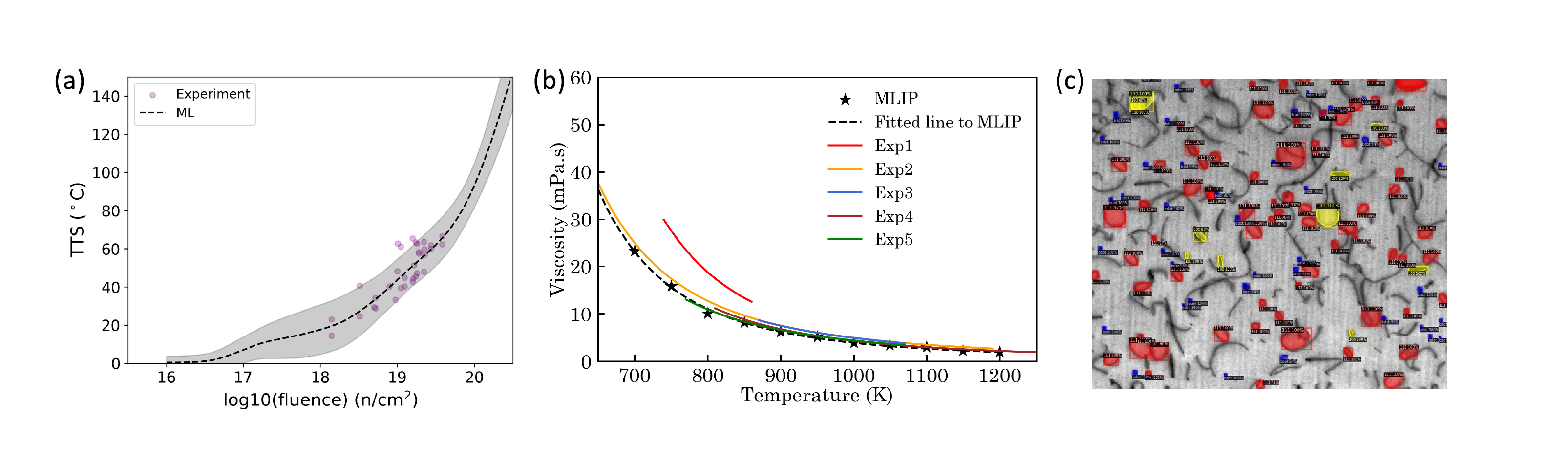
Exploring machine learning opportunities
The MS&E community is still in the early stages of developing the opportunities for ML, and we expect that continued improvements in ML and exploration in MS&E will lead to many further innovations. One exciting vision for the future of MS&E is what we call Integrated Computational and Materials Engineering (ICME) v2.0, where an artificial intelligence (AI) agent uses ML to guide autonomous experiments and computational modeling, and extract data from many varied sources.
This AI could then perform scalable autonomous materials discovery and optimization, dramatically accelerating our ability to improve human welfare by developing new materials.
References
- D. Morgan and R. Jacobs, “Opportunities and Challenges for Machine Learning in Materials Science”, in Annual Review of Materials Research, v.50 (2020).
- DOI: 10.1146/annurev-matsci-070218-010015
- Y. C. Liu, H. Wu, T. Mayeshiba, B. Afflerbach, R. Jacobs, J. Perry, J. George, J. Cordell, J. Y. Xia, H. Yuan, A. Lorenson, H. T. Wu, M. Parker, F. Doshi, A. Politowicz, L. D. Xiao, D. Morgan, P. Wells, N. Almirall, T. Yamamoto, and G. R. Odette, “Machine learning predictions of irradiation embrittlement in reactor pressure vessel steels,” npj Comput. Mater. v.8 (2022).
- DOI: 10.1038/s41524-022- 00760-4
- R. Jacobs, T. Mayeshiba, B. Afflerbach, L. Miles, M. Williams, M. Turner, R. Finkel, and D. Morgan, “The Materials Simulation Toolkit for Machine learning (MAST- ML): An automated open source toolkit to accelerate data-driven materials research,” Comput. Mater. Sci. v.176 (2020).
- DOI: 10.1016/j.commatsci.2020.109544
- S. Attarian, D. Morgan, and I. Szlufarska, “Thermophysical properties of FLiBe using moment tensor potentials,” J. Mol. Liq. v.368 (2022).
- DOI: 10.1016/j.molliq.2022.120803
- R. Jacobs, M. R. Shen, Y. H. Liu, W. Hao, X. S. Li, R. Y. He, J. R. C. Greaves, D. L. Wang, Z. M. Xie, Z. T. Huang, C. Wang, K. G. Field, and D. Morgan, “Performance and limitations of deep learning semantic segmentation of multiple defects in transmission electron micrographs,” Cell Reports Physical Science v.3 (2022).
- DOI: 10.1016/j.xcrp.2022.100876
- D. Morgan PM. Polak, “Extracting Accurate Materials Data from Research Papers with Conversational Language Models and Prompt Engineering,” arXiv:2303.05352 (2023)
- DOI: 10.48550/arXiv.2303.05352

This work is licensed under Creative Commons Attribution-NonCommercial-NoDerivatives 4.0 International.