Experts from IHE Delft Institute for Water Education and Eurecat, Centre Tecnològic de Catalunya, guide us through the use of machine learning tools and systems models to inform WEFE nexus policy formulation and impact assessment
In the July 2024 edition, the water, energy, and food (WEF) nexus was introduced as a coherent interconnected resource system that strongly interacts with ecosystems (to form the WEFE nexus) and supports societal development.
In the September 2024 edition, the NEXOGENESIS approach for stakeholder engagement and co-creation in a nexus context was outlined.
The WEFE nexus
The WEFE nexus is extraordinarily complex, with each sector interacting with the other sectors and being affected by ‘externalities’ such as the impacts of climate change and socio-economic developments, which modulate resource demand, consumption, and exploitation in doing so, degrading ecosystems. Impacts, actions, and changes in one part of this interconnected system have ‘ripple’ effects throughout the whole system. When considering the WEFE sectors, we cannot think about each in isolation without considering the impacts on the other sectors. A single-sector, silo approach to natural resource management is insufficient, though dominating.
However, aligning policy formulation such that policies and interventions are harmonious, working together rather than trading off against each other, is highly challenging. This is true even for one policy, let alone multiple policies that themselves may be interacting and have conflicting objectives across resource sectors. This is where systems modelling and machine learning can be leveraged to light up pathways in the dark, which is the focus of this third article.
The European Commission Horizon2020 research project, “Facilitating the next generation of effective and intelligent water-related policies utilising artificial intelligence (AI) and reinforcement learning to assess the water-energy-food-ecosystem (WEFE) nexus” (NEXOGENESIS), researches the WEFE nexus system in five case studies (Nestos-Mesta river basin, Bulgaria/Greece; Lielupe river basin, Lithuania/Latvia; Jiu river basin, Romania; Adige river basin, Italy; Inkomati-Usuthu Water Management Area, South Africa), focussing on how machine learning can be used to support policy analysis and decision support in the context of multiple resources and an uncertain future. In this vein, NEXOGENESIS explores the potential impacts of many policies being implemented across WEFE sectors to achieve multiple, sometimes conflicting, goals.
The crucial role of stakeholder engagement in identifying relevant policies and goals, as well as advising on model focus and aims, was outlined in the September 2024 edition. Following a series of stakeholder workshops, system dynamics models (Ford, 2010) were developed for each case study, being tailored to their needs and requirements. These models dynamically link the WEFE sectors and use state-of-the-art IPCC datasets to project resource trends to 2050 under four different future pathways. This is done as the direction of future development is unknown, and therefore, a set of plausible futures was chosen to reflect this uncertainty. Policies identified with stakeholders were integrated directly into these systems models. As such, the nexus-wide impacts of implementing a policy or policies can be assessed under different futures.
However, a huge challenge arises when trying to assess multiple policy combinations (even ten policies can be implemented in any combination, giving over 3.5 million combinations) and their impact on system pathways. Another challenge is to select amongst combinations which perform ‘the best’ relative to policy goals and objectives. It is in this exploration that machine learning techniques come into their own.
Machine learning for nexus policy recommendations
Nexus policy-making can be understood as an optimisation problem, where among the available policy instruments, a well-designed policy package must be carefully developed (i.e. optimised), considering many nexus goals and their associated trade-offs. Traditional decision-support tools for nexus policy-making often operate in silos, focusing on individual sectors or goals in isolation. This approach is insufficient to address the interdependencies and trade-offs inherent in the nexus. To overcome these shortcomings, innovative AI approaches have recently been explored within the nexus research community, incorporating multi-objective mechanisms into the decision-making process (UEN, Tinn- Shuan et al. 2018).
However, these approaches encounter challenges when several objectives are involved, and they often require significant computational resources, making real-time policy-package generation during stakeholder discussions impractical.
NEXOGENESIS surpasses these narrow and limited approaches by adopting a holistic view of the entire system, offering more comprehensive, integrated, and accessible machine learning-based solutions for nexus policy recommendation and policy-making.
Machine learning and, more specifically, Reinforcement Learning (Sutton, 2018) plays a pivotal role in NEXOGENESIS by navigating the complexities of interconnected resource systems within the WEFE nexus. These models can analyse a vast number of possible future policy scenarios – an effort that would take humans months or even years – and deliver tailored policy recommendations in seconds. These recommendations aim to provide decision support for achieving nexus-oriented objectives, such as optimising resource use or meeting sustainability goals.
Whether the goal is to conserve water, enhance energy efficiency, or increase food production, the machine learning-based decision support system evaluates trade-offs and synergies between these sectors, assessing countless potential policy combinations. It learns from experience by dynamically simulating various future scenarios and evaluating thousands, if not millions, of possible policy combinations. By continuously interacting with system dynamics models that simulate nexus impacts, for example, in climate change or socio-economic developments, the decision support system refines its recommendations, ensuring they remain relevant even in the face of uncertainty.
Empowering policymakers with tailored, real-world decision support
One of the key strengths of the NEXOGENESIS machine learning-based decision support system is its flexibility in providing various types of policy recommendations based on user requests from the stakeholder consultation processes. Users can select objectives that span multiple WEFE sectors. For instance, they may want to improve water management while also boosting energy efficiency, enhancing food production, and protecting ecosystems. The system then generates and recommends multiple sets of effective policy package options that balance these interconnected goals. Each recommendation includes detailed information on expected effects, providing clear summaries of how the policies will impact the WEFE nexus as a whole.
Furthermore, the decision support system ensures that its recommendations are grounded in real-world needs. Its objectives are derived from legal frameworks, international directives, and Sustainable Development Goals, and they are validated through the NEXOGENESIS co-creation process with stakeholders, which helps maintain real-world relevance and responsiveness.
Ultimately, it provides policymakers and stakeholders with rapid and intelligent policy recommendations that balance multiple, often conflicting objectives. Offering a comprehensive view of the WEFE nexus and its complex interactions enables more informed decision-making in an increasingly uncertain and interconnected world. Additionally, it helps to initiate and facilitate stakeholder dialogue on WEFE nexus interlinkages and policy impacts, ensuring that diverse perspectives are considered in the decision-making process. This positions it as a powerful tool for short- mid- and long-term sustainable resource management.
References
- Ford A. 2010. Modeling the Environment, 2nd ed. Island Press, Washington DC.
- Uen, T. S., Chang, F. J., Zhou, Y., & Tsai, W. P. (2018). Exploring synergistic benefits of Water-Food-Energy Nexus through multi-objective reservoir optimization schemes. Science of the Total Environment, 633, 341-351.
- Sutton, R. S. (2018). Reinforcement learning: An introduction. A Bradford Book.
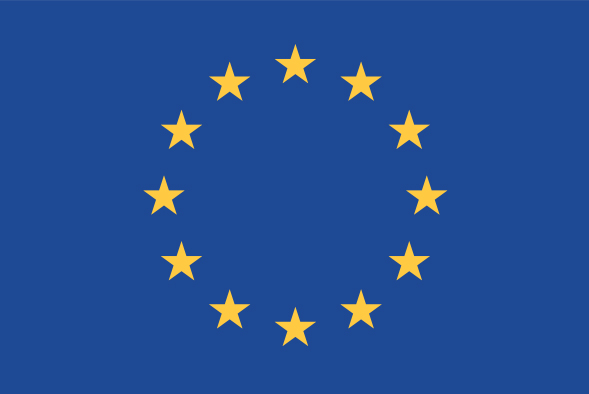