Matthew Shew, Amit Walia, and Craig A. Buchman highlight that the significant variability in speech perception among cochlear implant users can be addressed by using a multi- faceted approach that incorporates emerging technologies like machine learning and artificial intelligence to improve outcome prediction models
Cochlear implants (CIs) represent one of the most successful neural prostheses, providing discrete frequency and intensity electrical stimulation to restore hearing, improve speech understanding, and enhance quality of life. Despite their transformative impact, a significant challenge remains: the substantial variability in speech perception outcomes among CI users. (1) While current methodologies excel at identifying individuals with severe hearing loss and, thus, strong CI candidates, they fall short in predicting the degree of benefit each recipient will gain from the device. This limitation hinders personalized care and underscores the need for more advanced predictive tools.
At Washington University (WashU), our CI research programs are addressing this challenge through a multi-faceted approach aimed at refining CI outcome prediction models. Traditional preoperative variables, such as demographic data and hearing history, have shown limited utility in predicting outcomes, prompting us to explore alternative strategies. (2-4) For instance, electrocochleography (ECochG) techniques, which measure cochlear health, have been shown to account for 40–50% of the variability in CI performance. (2-4) Additionally, leveraging real-time electrophysiologic measurements enables us to develop patient-specific electrode mapping and programming strategies, further tailoring the CI experience. (5)
The next frontier in our work is the integration of big data and artificial intelligence (AI) technologies, such as machine learning and deep learning, into CI healthcare. Unlike traditional regression models that focus on single or limited features to explain variability, AI offers the ability to analyze complex, multi-dimensional datasets without being constrained by linear relationships, data source, or data type (Figure 1). These capabilities allow AI to uncover patterns and interactions across diverse clinical and biological inputs that traditional methods might overlook.
The intersection of auditory system-level pathophysiology and CI outcomes presents an ideal landscape for applying AI-based methods. By integrating heterogeneous datasets, including ECochG metrics, cognitive assessments, imaging, demographic, and surgical variables, AI has the potential to improve risk stratification and outcome prediction in ways that were previously unattainable. This shift from single biomarker reliance to holistic, AI-driven modeling brings us closer to achieving true precision medicine in cochlear implantation at WashU, ultimately enhancing patient care and optimizing CI performance for every individual.
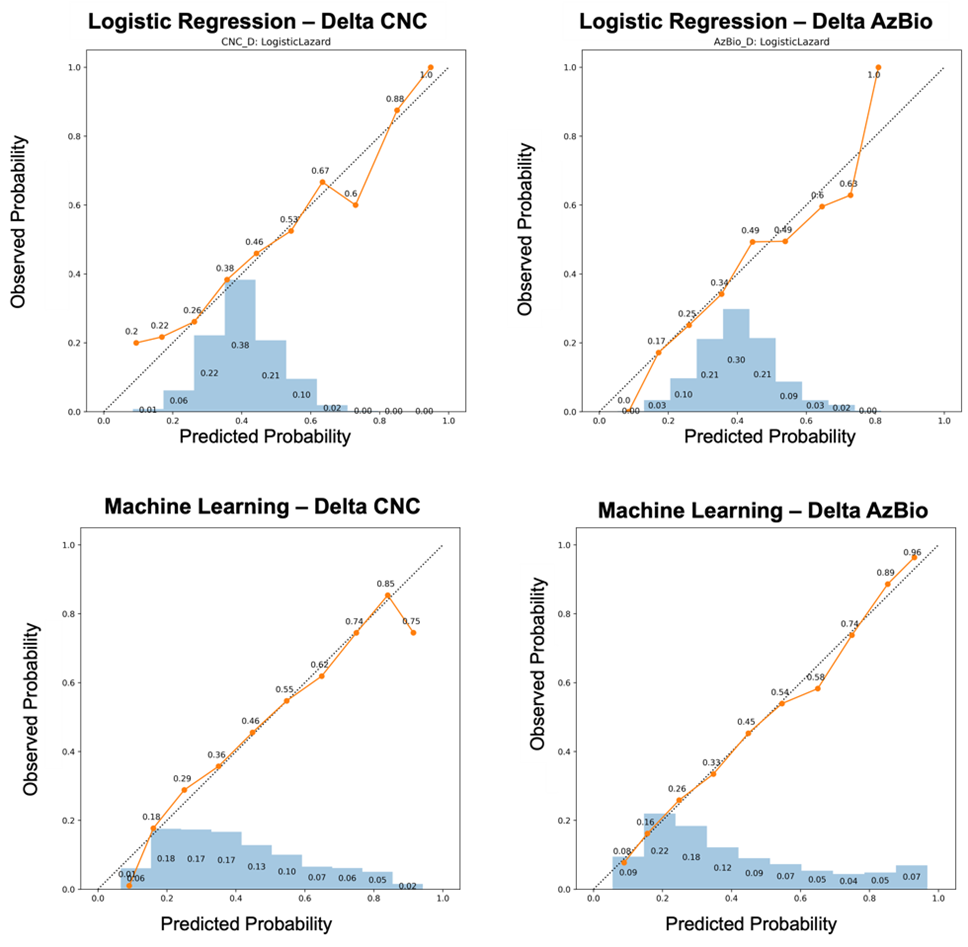
Potential of machine learning CI prediction modeling
At WashU, we utilized one of the largest CI datasets to date, encompassing over 2,200 CI recipients, to predict six-month CNC word and AzBio sentence scores based solely on preoperative features.
To assess the added value of ML methods, we compared their performance to traditional regression models, benchmarked against a landmark study by Lazard et al. (2012). (6) Our analysis focused on calibration curves and accuracy heatmaps, evaluating the models’ ability to predict performance quintiles, with an emphasis on distinguishing top and bottom performers. The findings highlight the significant potential of ML-based models to uncover novel subcategories of CI performance compared to conventional regression approaches.
Calibration curves demonstrated the enhanced reliability and predictive value of ML-based models (Figure 2). Unlike standard regression techniques, which concentrated over 69% of predictions within the 0.30–0.60 probability range (akin to flipping a coin), ML models provided a broader distribution of probabilities. For instance, ML-based models produced a greater proportion of predictions at both low (e.g., 0.10 or 0.20) and high (e.g., 0.80 or 0.90) probability ranges, enabling a more clinically actionable framework (Figure 2). This broader probability distribution supports superior calibration and highlights the potential of ML to offer predictions with higher clinical relevance.
To further explore ML-based models as decision support tools within CI healthcare pathways, we constructed accuracy heatmaps to visualize their ability to predict performance quintiles for delta (Δ) AzBio sentences (Figure 3). By prioritizing predictions in the top third of probabilities, we leveraged ML models’ superior calibration to provide a clinically focused decision model at the expense of only being applicable to one third of patients. This approach demonstrated that ML methods outperformed traditional regression in identifying both the lowest and highest category performers. For example, ML models achieved a 63% accuracy in predicting the lowest quintile of ΔAzBio performance (≤20th percentile; ΔAzBio ≤13%), compared to 33% accuracy with logistic regression models.
Finally, ML-based models showed innovation in recognizing near misses, such as patients falling within the second quintile, offering insights into subgroups of CI performance not previously identifiable. For instance, when predicting a patient to be in the lowest quintile for ΔAzBio performance, ML models achieved 63% accuracy while also capturing 26% in the second quintile, collectively identifying an 89% likelihood of achieving ΔAzBio ≤32%. These results underscore the transformative potential of ML in CI prediction modeling. By enabling more accurate stratification of patient outcomes, ML offers a pathway to personalized CI care, improving clinical decision-making and paving the way for tailored interventions that enhance patient outcomes.
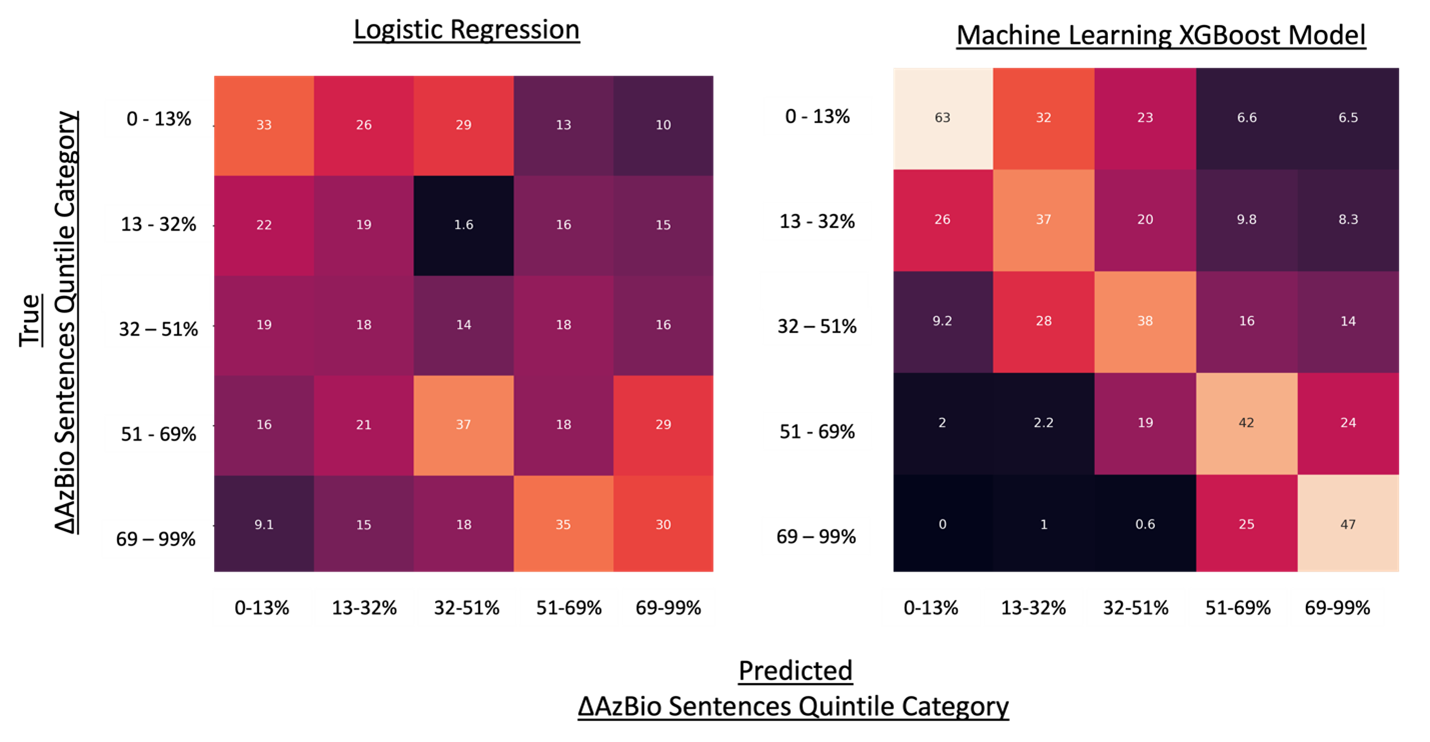
Looking to the future
In the most recent series (see futher reading), our CI research program at WashU has adopted a comprehensive, multi-pronged approach to predicting CI performance for any individual recipient. Our findings underscore that an ideal prediction model must integrate multiple biomarkers, including cochlear health (ECochG-TR), cognitive function, frequency-to-place mismatch, and patient-specific factors, alongside traditional preoperative variables. (7)
By building some of the most extensive and detailed CI datasets to date incorporating these novel features, we aim to harness AI-based methods to refine our understanding and prediction of performance subcategories for personalized patient counseling, designing and recommendation of auditory rehabilitation, and consideration for device-related underperformance (e.g., mapping, troubleshooting, early device failure), and ultimately proper stratification for CI clinical trials. This work holds the potential to revolutionize patient care, delivering truly personalized predictions and optimizing CI outcomes for every patient.
References
- Shew M, Herzog JA, Buchman CA. For Whom Do Cochlear Implants Work Best? JAMA Otolaryngol Head Neck Surg. Jul 1 2020;146(7):603-604. doi: https://doi.org/10.1001/jamaoto.2020.0678
- Walia A, Shew MA, Kallogjeri D, et al. Electrocochleography and cognition are important predictors of speech perception outcomes in noise for cochlear implant recipients. Sci Rep. Feb 23 2022;12(1):3083. doi:https://doi.org/10.1038/s41598-022-07175-7
- Walia A, Shew MA, Lee DS, et al. Promontory Electrocochleography Recordings to Predict Speech-Perception Performance in Cochlear Implant Recipients. Otol Neurotol. Sep 1 2022;43(8):915-923. doi:https://doi.org/10.1097/mao.0000000000003628
- Walia A, Shew MA, Lefler SM, et al. Factors Affecting Performance in Adults With Cochlear Implants: A Role for Cognition and Residual Cochlear Function. Otology & Neurotology. 9900;
- Walia A, Ortmann AJ, Lefler S, et al. Electrocochleography-Based Tonotopic Map: I. Place Coding of the Human Cochlea With Hearing Loss. Ear Hear. Sep 5 2024;doi:https://doi.org/10.1097/aud.0000000000001579
- Lazard DS, Vincent C, Venail F, et al. Pre-, per- and postoperative factors affecting performance of postlinguistically deaf adults using cochlear implants: a new conceptual model over time. PLoS One. 2012;7(11):e48739. doi:https://doi.org/10.1371/journal.pone.0048739
- Dang S, Kallogjeri D, Dizdar K, et al. Individual Patient Comorbidities and Effect on Cochlear Implant Performance. Otol Neurotol. Feb 29 2024;doi:https://doi.org/10.1097/mao.0000000000004144
Further Reading
- Predicting cochlear implant performance: Impact of demographic, audiologic, surgical factors, and cochlear health
- Cognitive function and electrode mapping’s role in cochlear implant performance
- Hearing and auditory research for cochlear implant outcomes