Founder and President of InsideOutBio, Alan Herbert, explores the evolving understanding of genome information storage, and the significance of repetitive sequences called flipons in genome training. These flipons can alter their shape without breaking DNA and are vital in cell biology, especially in responding to environmental stress
Our view of how genomes store information has rapidly changed. In the classical view, codons contain the important content. Each codon consists of three DNA bases uniquely mapped to an amino acid. The order of codons gives the position of amino acids in the protein product that determines its function. Mutations that change codons or their expression then cause disease. In this view, the repetitive sequences that comprise over 50% of the human genome are just a nuisance. They cause genomic instability by frequently breaking and disrupting DNA repair. However, some repeats are useful. For example, telomeric repeats help protect chromosome ends and prevent senescence, while those at centromeres play a role in cell division. Otherwise, most repeat sequences are confined by nucleosomal proteins to limit the harm that they can cause. Recently, a more nuanced understanding of how genomes work has emerged. With this new focus, repeats that dynamically change their conformation in a programmable manner play important roles in cell biology. This class of repeats is called flipons. They enable genomes to learn and optimize cell responses to environmental stressors.
Two factors are driving this radical change in perspective. One is the realization that DNA and RNA can fold in different ways. In addition to the Watson and Crick right-handed double helix, DNA can twist to form left-handed Z-DNA or adopt three and four-stranded structures (Figure 1). The flipons morph their conformation without breaking DNA. Only an input of energy is required to power the flip. The other major change in perspectives arises from the data deluge delivered by the human genome project and the technologies it spawned. It was recently estimated that fifty quintillion biological bytes are generated annually, with the production rate still increasing.
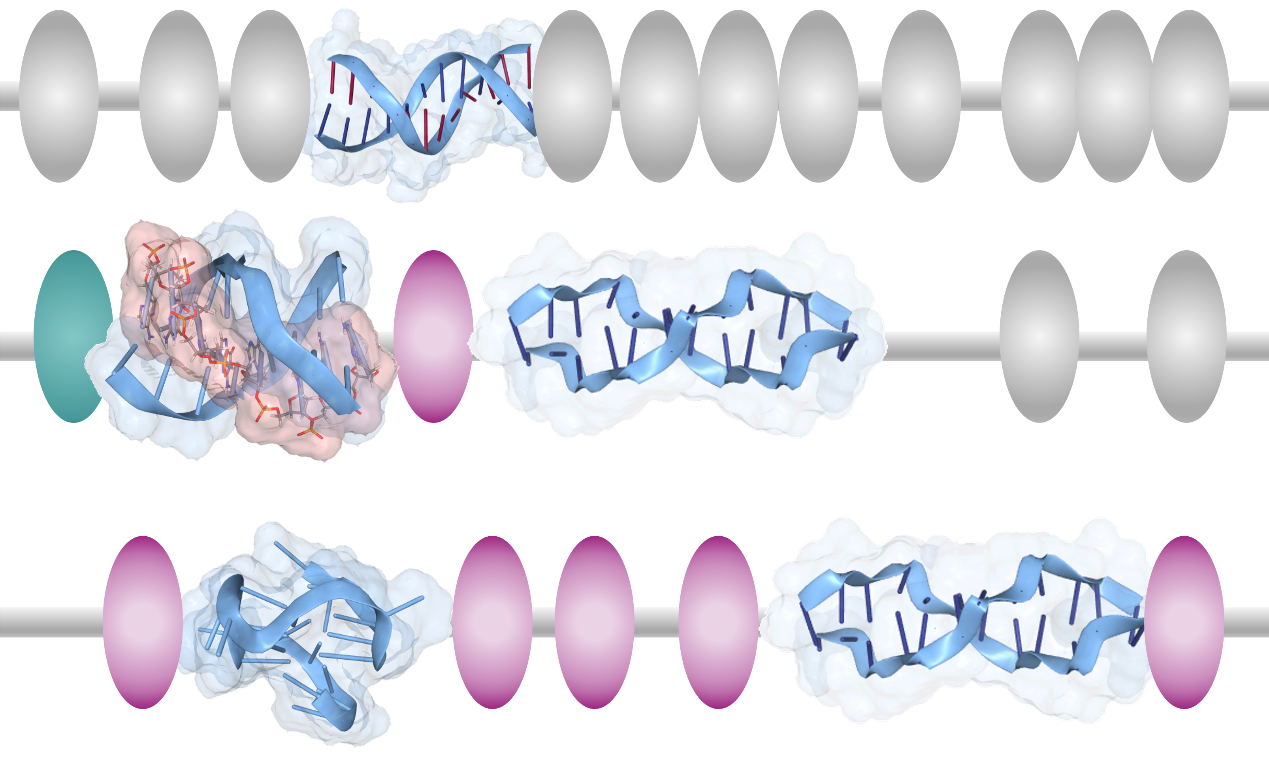
What can you do with all this data?
This year’s Nobel Prize in Chemistry exemplifies the usefulness of collecting information on such a vast scale. The problem the prize winners solved was predicting protein folding into a functional form with only the coding sequence available. They trained two models: One was based on how proteins evolve over time, and the other on a small sample of proteins with known structures. As they learned independently, the models used their predictions to enhance both their own performance and that of the other model. The approach identified the most informative set of residues in each protein sequence by detecting amino acids that are close in evolutionary and structural space. Once optimized, the two expert models assisted in training a production model to predict an unknown protein’s structure de novo, with further improvements in accuracy achieved by fine-tuning model parameters using other known protein structures. The protein structure predictions generated are now recognized to have a high probability of experimental validation. These predictions enhance our understanding of how drugs target specific proteins and are now aiding in the design of better drugs.
Similar information-dense maps are available for genomes. The maps show where genes start and finish and whether the RNA they encode is transcribed in a certain cell or context. Maps are also available that locate the epigenetic tags on each chromosome. The tags vary with cell and tissue type. They signal whether a gene is silenced, poised while awaiting further instructions, deployed and ready to fire, or constitutively active.
Flipon maps for different DNA conformations are now available
Those for Z-DNA and four-stranded folds, G-quadruplexes, are enriched in regions promoting gene expression – their conformation changes during transcription. G-quadruplexes provide a mechanism to load and lock transcription factors. The primed promoters only fire when the signal to start making RNA is given. Z-DNA ensures promoter reset to allow a new round of transcription. A different three-stranded flipon, called a triplex, helps open up the regions where other flipons do their acrobatic flips. Sometimes, triplexes are programmed using an RNA to target a specific DNA sequence. The RNA docks to the DNA to form a three-stranded triplex fold (red strand in Figure 1). The triplex then helps position nucleosomes on chromosomes to create a nucleosome-free region (NFR) of DNA. Other flipons within the NFR are then free to change conformation. These transitions can be programmed by sequence-specific RNAs and proteins. The nucleosome beads on a DNA string resemble an abacus. Both calculations are based on bead spacing (Figure 2). One difference is that the flipon conformation varies with context, as does the probability of a flip.
We know that cells adopt multiple states according to their stage of differentiation. The cell state also varies over time with exposure to hormones, other signaling molecules, and pathogens. Cells adapt to these changes. At one extreme, immune cells learn by mutating antibody and antigen receptor genes to improve their binding specificity for an infectious agent. However, such changes are irreversible. So, can cells learn dynamically and reversibly? Flipons enable such outcomes. The cell responds by altering the probability that a flipon will flip in response to a particular input. Cells can write specific epigenetic marks on chromatin that enhance or suppress flipon transitions from one state to another (Figure 2). The tags can be written by complexes that bind selectively to one flipon conformation or the other. The tags change the probability of a flip the next time a cell receives the same input. Cells that optimize responses will undergo selection to produce progeny that preferentially populate a tissue.
The strategy works because flipon conformations are probabilistic, with no two cells having the same flipon settings as their genetic twins. The new field of p-bit computing is based on similar probabilistic reasoning. The algorithms deployed can solve some problems more efficiently than is possible with deterministic binary computers while avoiding the complexity of a quantum computer. Here, the calculation starts with the answer. The challenge is to find how that answer was derived. The p-bit computer uses transistors to perform calculations but exploits random noise to vary the initial probability that the transistors are in an on or off state. After each reiteration, the likelihood that transistors are on or off varies until the ensemble collectively yields the correct output. Once the circuit delivers the desired answer, the registers that internally store the transistor states are read out to give the settings that produce the result. Analogously, flipon probabilities can undergo adjustments in a cell to find a state that offsets environmental perturbations to maintain the cell state. These factors are what we hope to capture with our vast collection of data maps.
Journal of the Royal Society
A paper just published in the Journal of the Royal Society Interface addresses how flipons enable genomes to learn in more detail. The manuscript, authored by Alan Herbert, describes the biological, statistical, and computational underpinnings of flipon-based programming. Included in the paper is a practical guide on the use of large language models to train genomes. The main goal of the work is to reset states that produce disease by reprogramming flipons within the cell. Previously, the genome-wide reset of flipons has been used to reprogram a differentiated cell back to a stem cell. The computational models will allow a more tailored approach, allowing the design and evaluation of sequence-specific RNAs and small drugs that target particular flipon combinations. We now have the data to guide the discovery of factors that lead to a particular outcome. However, we still have much to learn about how genomes learn.
References
- Herbert A. Flipons and the logic of soft-wired genomes. 1st ed. Boca Raton: CRC Press; 2024.
- Herbert A. Flipons Enable Genomes to Learn by Intermediating the Exchange of Energy for Information. The Journal of the Royal Society Interface. 2025; in press.