Marcel van Gerven, a Professor of Artificial Cognitive Systems at Radboud University, discusses how his team’s research on AI models, algorithms, and demonstrators could serve as a blueprint for the semiconductor manufacturing industry
Artificial Intelligence (AI) is a key enabling technology that, when used responsibly, can bring about significant positive societal impact. One of the initiatives to foster this positive impact is the Dutch ROBUST ‘Trustworthy AI-based Systems for Sustainable Growth’ program. This ten-year Long Term Program (LTP) is a human- centered research program in AI that brings together knowledge institutes, industry, governmental organizations, and societal partners to develop AI-based methods and tools designed to create social impact and promote sustainable growth (Figure 1).
Sustainable and responsible AI solutions in semiconductor manufacturing
ROBUST’s research agenda focuses on the principles underlying trustworthy AI to realize AI’s potential to create social impact. Six dimensions of trustworthy AI, namely accuracy, explainability, reliability, repeatability, resilience, and safety, guide the development of algorithms addressing specific objectives motivated by the UN’s Sustainable Development Goals (SDGs).
One of the key areas in which AI can achieve important breakthroughs is semiconductor manufacturing, as this is a large industry with a significant global impact. To achieve such breakthroughs, AI researchers from the Donders Institute at Radboud University, Nijmegen, the Netherlands, have joined forces with ASMPT as an industrial partner to establish the ASMPT ICAI Lab for Semiconductor Manufacturing as part of the ROBUST program. This ICAI lab focuses on the development of sustainable and responsible AI solutions in the semiconductor manufacturing industry, contributing to SDG 9: Industry, Innovation, and Infrastructure and SDG 12: Responsible Consumption and Production. We envision that embracing AI methods will improve the accuracy and reliability of semiconductor manufacturing, resulting in more efficient industrial processes and less waste of natural resources. This is in line with ASMPT’s 2035 net zero objective.
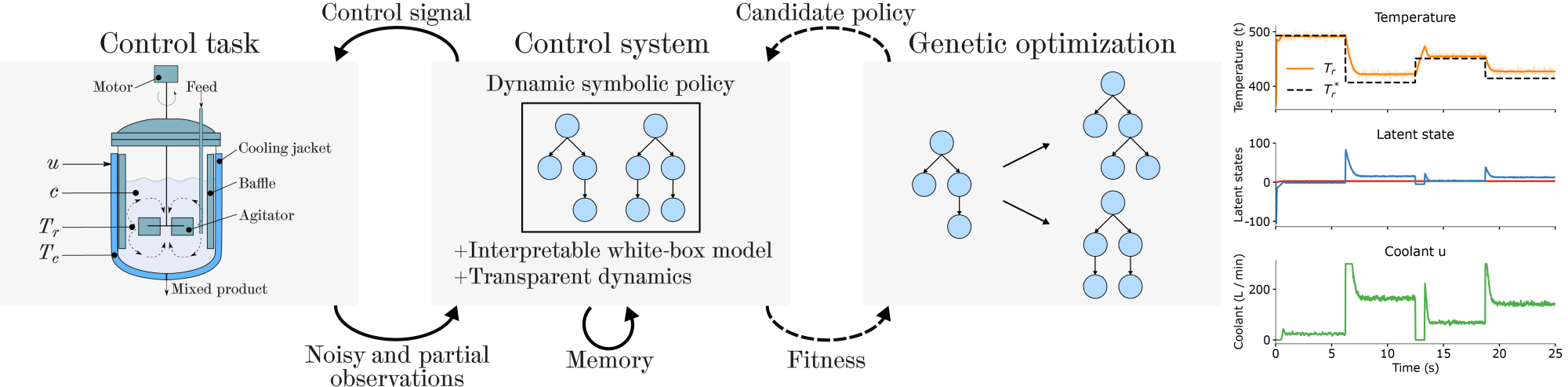
In advanced micro and nanoscale manufacturing, controlling the quality of produced devices presents major challenges for conventional automated inspection and process control techniques. The production of modern microelectronic devices, with its high levels of 3D integration and shrinking structure sizes, requires new approaches to sensing-based inspection, prediction, and control. While the recent use of deep learning already offers new possibilities for microscale defect detection of semiconductor manufacturing equipment, even such a powerful tool, in its current form, shows serious limitations when deployed in complex production environments. Techniques that are energy-efficient and operate in real-time with less (or no) supervision are the only viable solution for high- throughput / high-precision industries. Furthermore, the developed approaches should be trustworthy to ensure value-aligned deployment in the real world. Motivated by these observations, the ASMPT lab aims to develop computational methods and workflows that process the readily available sensory streams for both low-level physical control and high-level decision-making.
PhD students in the ASMPT lab focus on several AI approaches that each contribute to these aims. The first objective is to develop more efficient deep learning methods that afford real-time processing while reducing the data and energy needs of learning and inference. A second objective is the development of advanced Bayesian optimization methods and model predictive control methods that allow automatic optimization of industrial processes. A third objective is the development of new embedded AI platforms using field-programmable gate arrays that allow for fast machine learning on the edge. This enables the integration of AI methods into industrial workflows without needing to resort to cloud computing. A fourth objective is to embrace neuromorphic computing as a computational paradigm. This event-driven, brain-inspired approach holds the promise of much faster and more energy-efficient AI processing.
As a concrete example of one of the early breakthroughs in our lab, consider the optimal control of industrial processes. To this end, we developed a genetic programming approach that optimizes neural differential equations that learn to control a plant. Figure 1 provides a high-level overview, demonstrating the control of a continuous stirred tank reactor. We show that the resulting controllers are effective (as they learn to control the plant), efficient (as they consist of a small number of free parameters), transparent (since we can interpret the dynamics of the learnt controller), and resilient (since sudden changes in the plant can be accommodated by the controller).
In short, the ASMPT lab focuses on improving the robustness of high-throughput streaming data inference and control systems in the chip manufacturing industry (extrinsic trustworthiness). Furthermore, it will contribute to improving the explainability of decisions made by these systems (intrinsic trustworthiness). As such, AI models, algorithms, and demonstrators developed in the lab may act as a blueprint for the semiconductor manufacturing industry as a whole. Currently, stakeholders from Radboud University and the regional semiconductor manufacturing ecosystem are intensifying their collaboration to further accelerate impact. Here, we foresee that particularly research at the intersection of the natural sciences (physics, chemistry) and Artificial Intelligence (machine learning, neuromorphic computing) will bring about important advances, such as advanced metrology and process control, as well as novel unconventional computing paradigms such as neuromorphic computing, spintronics and photonics that define the future of semiconductor manufacturing.
The semiconductor manufacturing use case demonstrates one of the ways in which AI can bring about positive change. In the same vein, we expect that many domains in science, industry, and society can be aided by AI. This ranges from improving the efficiency and quality of healthcare to mitigating the environmental crisis we are faced with. At the same time, we should not be blind to the negative impact of AI, ranging from disrupting political processes and the development of autonomous weapons to worsening the global energy crisis. These observations are not new. Already in the late 1940s, Norbert Wiener, the founder of cybernetics, from which AI originates, talked about the potential societal benefits of automation, as well as how to mitigate its dangers. As stated by Wiener: The answer, of course, is to have a society based on human values other than buying or selling. Which way the dice rolls is our shared responsibility.
This publication is part of the project ROBUST: Trustworthy AI-based Systems for Sustainable Growth with project number KICH3.L TP.20.006, which is (partly) financed by the Dutch Research Council (NWO), ASMPT, and the Dutch Ministry of Economic Affairs and Climate Policy (EZK) under the program LTP KIC 2020-2023. All content represents the opinion of the authors, which is not necessarily shared or endorsed by their respective employers and/or sponsors.

This work is licensed under Creative Commons Attribution-NonCommercial-NoDerivatives 4.0 International.