Predicting Response to Neoadjuvant Chemotherapy Using Tumor Vasculature Characteristics
Ursa Brown-Glaberman, MD – University of New Mexico Comprehensive Cancer Center, Albuquerque, NM 87131, USA
Terisse A. Brocato, PhD – Department of Chemical and Biological Engineering and Center for Biomedical Engineering, University of New Mexico, Albuquerque, NM 87131, USA
Reed Selwyn, PhD – Department of Radiology, University of New Mexico, Albuquerque, NM 87131, USA
Zhihui Wang, PhD – Mathematics in Medicine Program, Houston Methodist Research Institute, Houston, TX 77030, USA
Vittorio Cristini, PhD – Mathematics in Medicine Program, Houston Methodist Research Institute, Houston, TX 77030, USA
Breast cancer is a model disease in precision oncology, leading the way in the development of both targeted therapy and prognostic and predictive biomarkers. However, predictive biomarker based treatment selection remains an elusive goal in the management of many women with this disease. Our group has developed a modeling tool to predict the likelihood of response to neoadjuvant chemotherapy using patient specific tumor vasculature biomarkers. Here, we describe a novel integrated study based on a mathematical model utilizing tumor vasculature characteristics paired with patient data analysis to predict response to neoadjuvant chemotherapy in women with high risk hormone receptor positive, HER2 negative early stage breast cancer.
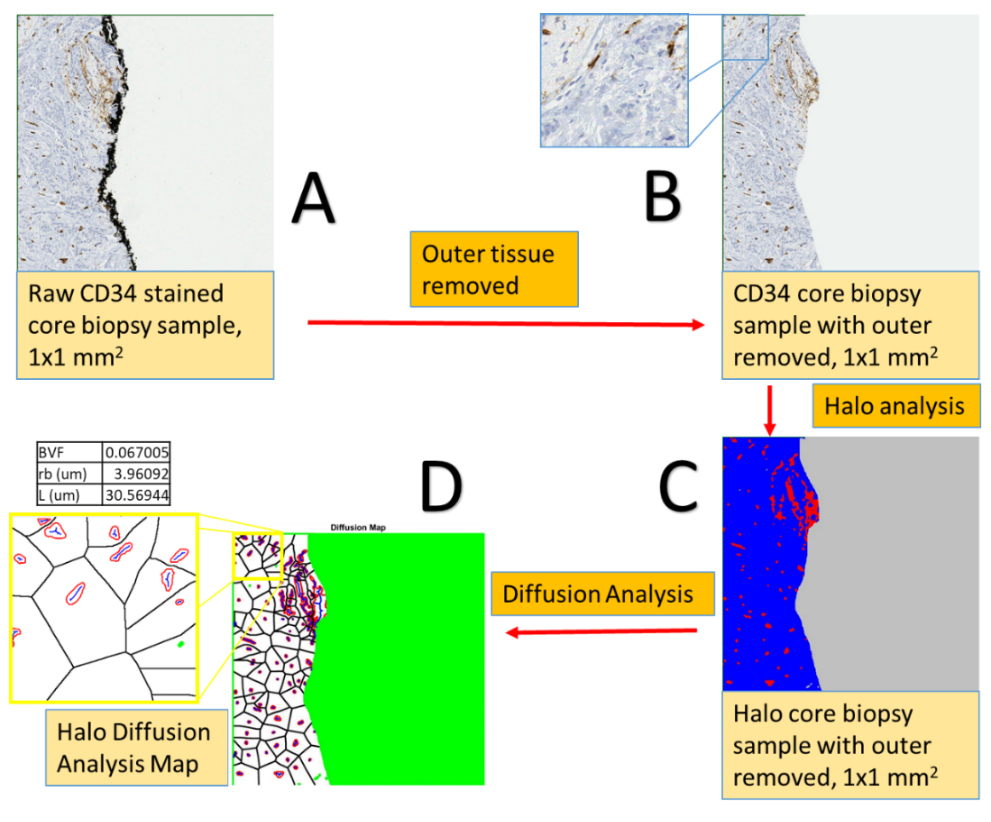
We have pioneered a semi-automated analysis method that allows for increased measurement accuracy and rapid throughput in rendering model predictions, with hundreds images analyzed for each patient (Figure 1). First, we applied a histology-based model to primary resected breast cancer tumors. Second, we then evaluated a cohort of patients undergoing neoadjuvant chemotherapy, collecting clinically relevant data including pre- and post-treatment pathology specimens, and dynamic contrast-enhanced magnetic resonance imaging. We correlated predicted outcome based on our model with actual clinical outcome, including rate of complete pathologic response (pCR) following neoadjuvant chemotherapy. We found that core biopsy samples of primary breast tumors can be used with acceptable accuracy to determine histological parameters representative of the whole tissue region. We further correlated response to neoadjuvant chemotherapy with the pretreatment tumor vasculature biomarkers and model parameters. Analysis of histology parameters, specifically radius of drug source divided by diffusion penetration distance (L/rb), a normalization penetration distance, and blood volume fraction (BVF), provides a separation of patients obtaining a pathologic complete response (pCR) and those that do not, with 80% accuracy (P = 0.0269) (Figure 2). With this predictive model, we are able to evaluate primary breast tumor vasculature biomarkers in a patient specific manner, thereby allowing a precision approach to breast cancer treatment.
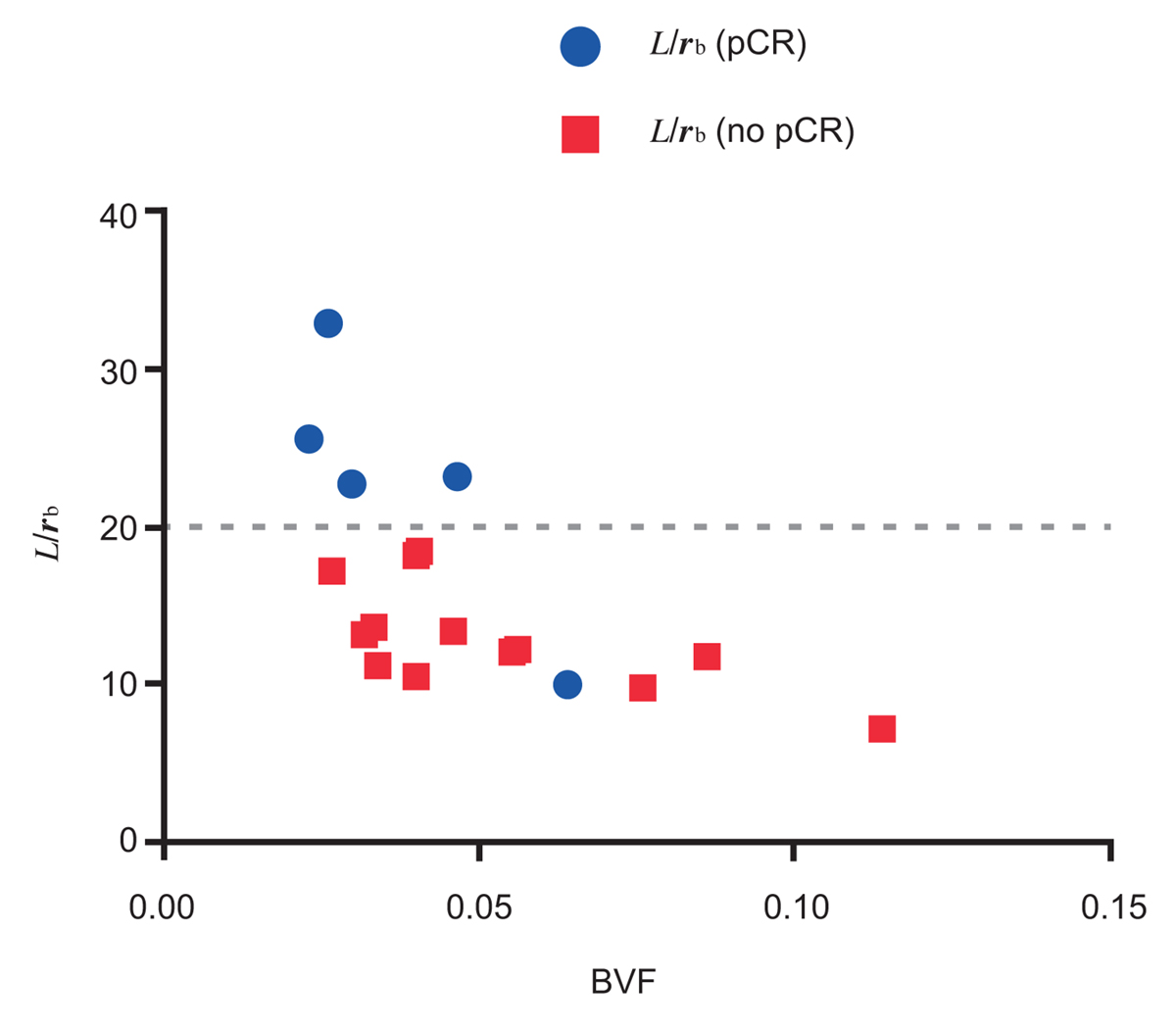
Please note: This is a commercial profile
Ursa Brown-Glaberman, MD
University of New Mexico Comprehensive Cancer Center,
Albuquerque, NM 87131, USA
Terisse A. Brocato, PhD
Department of Chemical and Biological Engineering and Center for Biomedical Engineering,
University of New Mexico,
Albuquerque, NM 87131, USA
Reed Selwyn, PhD
Department of Radiology,
University of New Mexico, Albuquerque, NM 87131, USA
Zhihui Wang, PhD
Mathematics in Medicine Program,
Houston Methodist Research Institute, Houston, TX 77030, USA
Vittorio Cristini, PhD
Mathematics in Medicine Program,
Houston Methodist Research Institute, Houston, TX 77030, USA
Tel: +1 505 934 1813
vcristini@houstonmethodist.org
www.houstonmethodist.org/faculty/vittorio-cristini/
ISI Highly-Cited Researchers in Mathematics: http://highlycited.com
Google scholar: https://scholar.google.com/citations?user=uwl5tw0AAAAJ&hl=en