Shai Gordin, Senior Lecturer at Digital Pasts Lab, Ariel University in Israel, provides intriguing insights about reading & deciphering ancient writing systems using AI
Can computers read ancient language systems better than experts? And why would we want AI to do that? How exactly do computational models read texts?
Computational methods to study ancient texts & scripts
There are multiple benefits of using computational methods for studying ancient texts and scripts, like Egyptian hieroglyphs or Mesopotamian cuneiform writing. Imagine all ancient texts were available with an effective search engine. You can search for specific words or sign sequences or even general keywords. The benefits of searchable databases for texts in hieroglyphic, cuneiform, or any of the alphabetic scripts of the ancient world are already well established, with websites like Trismegistos, the Cuneiform Digital Library Initiative, Hethitologie Portal Mainz, or the Comprehensive Aramaic Lexicon Project, to name a few.
Another benefit is consistency in the work process. Often historical research involves reading substantial amounts of texts and extracting relevant information. In other words, it is a task of meticulous close reading, later synthesised by an expert. Doing this process digitally helps to maintain consistency and accuracy. In fact, saving pieces of data in digital format is not at all different from traditional methods of card-file catalogues. Instead of storing small papers with pertinent information in specific boxes, they are stored digitally in files that are like cabinets: their structure, which is easy for a computer to process, is like folders within folders, which can easily take you to the information you are looking for. The important difference is that when these folders and cards are digital, you can easily rearrange them, visualise, explore them and reuse them in ways that were not possible before.
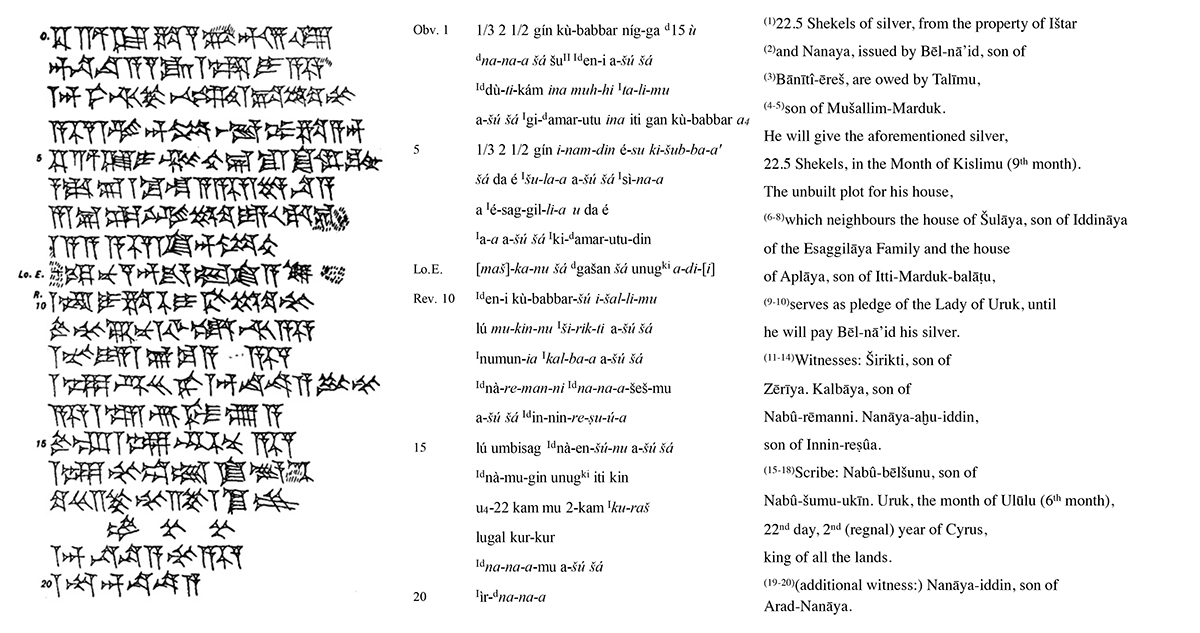
Computational text analysis is far from simply translating analogue methods to digital ones. Using computational methods with ancient texts and scripts can open new avenues of research that were completely impossible before. Computers understand text very differently from humans. A computer often needs to translate words into numbers or vectors, and then it can analyse lexical similarities and meanings using mathematical formulae. While this practice is completely foreign to traditional textual analysis, it opens an opportunity to view texts from a new perspective. This is just one example of many possibilities. Moving in a digital direction also provides opportunities for interdisciplinary research on an unprecedented scale. Think of the card-file catalogue example from above. When it is analogue, these boxes are of use to a limited number of scholars with access, and they cannot be connected to catalogues of other scholars from the same or adjacent fields. But if they are digital, these files can be shared, compared, and joined together through the semantic web.
Natural language processing (NLP) models
While the digital corpora of ancient texts are constantly growing, many are still far from full digitisation. Reading ancient documents is difficult, the decipherment takes time, the size of the corpus is substantial and may include several ancient languages. Natural language processing (NLP) models can aid these tasks. NLP is a subfield in computer sciences and linguistics that studies and develops methods for computers to understand and analyse human languages. The best models are often neural networks, advanced machine learning (ML) models whose mechanism attempts to imitate the workings of the human mind, ergo, their name.
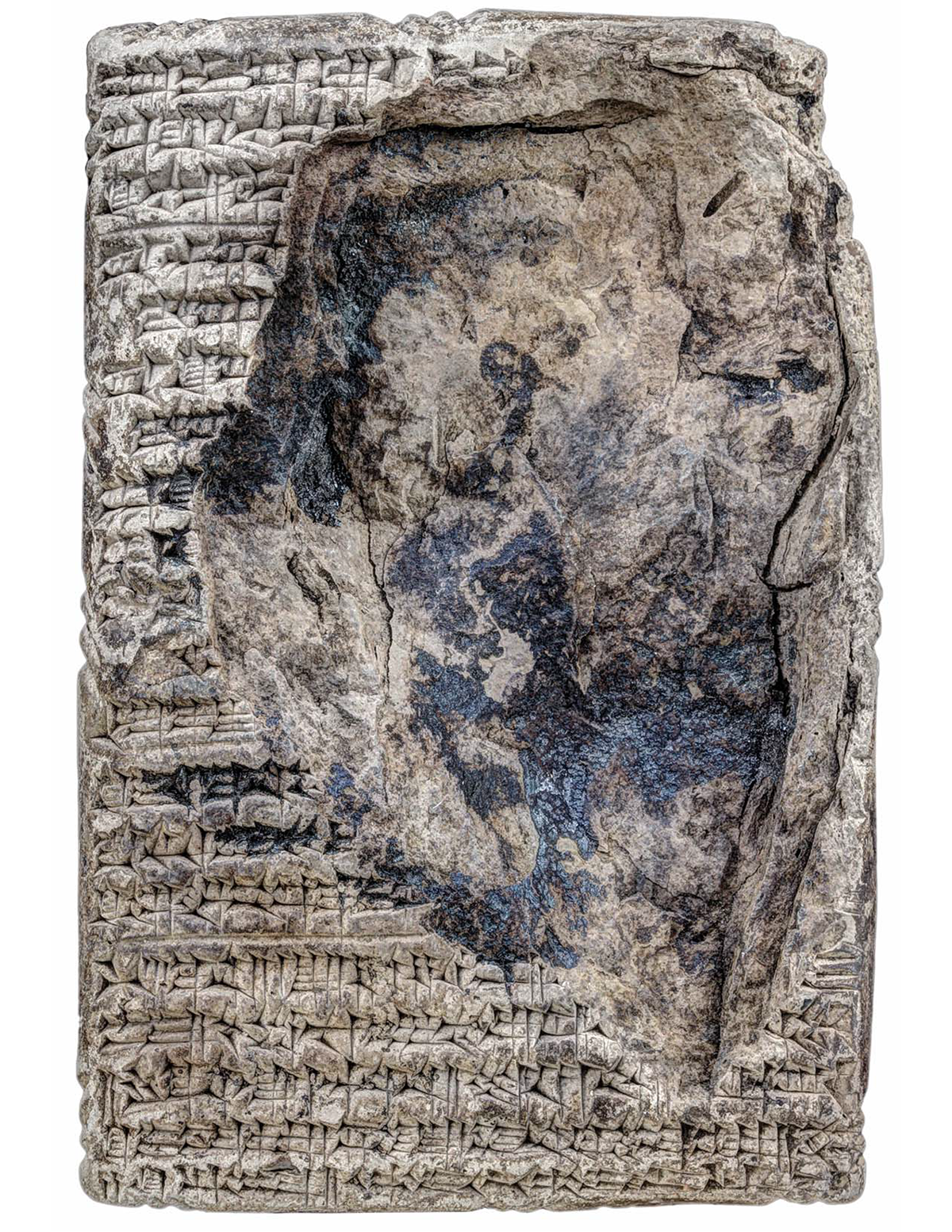
(Yale University, New Haven, CT).
But while a human does not need to see millions of images of cats and dogs to differentiate between the two, neural networks do. This is not a problem when training models on the English language, for example. It is easy to extract tens of millions of words in English from the internet. Many ancient languages, however, fall under the category of low-resource languages – these have a limited number of examples, and some of them, like Akkadian, also have more complex morpho-syntactic structures. The upside of some text genres, even historical ones, is that many are formulaic and repetitive, unlike modern languages which are highly variable in nature. This counteracts, to a certain extent, the sparseness of available texts.
One of the common tasks in NLP is restoring masked words in a sentence – a task which, in practicality, is equivalent to restoring broken passages in ancient texts. Based on the complete sentences which the model has seen, it can predict how to restore fragmentary sentences. The more examples it will see, the better the results are. My research team at the Digital Pasts Lab, as well as another group from the Hebrew University, have shown the effectiveness of NLP models for restoring fragmentary cuneiform tablets written in Akkadian.
A related NLP task is predicting sequences of words or in the case of cuneiform, which can also be signs. By using Neo-Assyrian royal inscriptions and their equivalent Unicode cuneiform glyphs, it was possible to effectively train a model to provide transliteration and segmentation of cuneiform signs. This was performed using neural networks, as before, and statistical models, which look at the frequency in which certain sign readings follow each other. The above tasks could be performed by training models on existing digital texts. The greatest challenge, however, lies in optical character recognition (OCR), the visual identification of cuneiform signs; be that from drawings of cuneiform tablets, 2D images or 3D scans.
The future of reading ancient texts and scripts
The success of this kind of research allows us a glimpse into what will reading ancient texts and scripts look like if computers can perform all tasks, from visual recognition to transliteration and segmentation, even translation. Can we then say that the models are reading texts like scholars? Not yet. It is important to remember that even when the models perform well, they do not perform perfectly. There is always a margin of error. Besides the fact that there is not necessarily an ultimate truth when reading and reconstructing ancient texts – some issues are open to interpretation.
The scholar, then, needs to guide the models, correct them and analyse their results from a humanistic perspective. The combination of human and machine-based approaches will offer fresh perspectives on classical problems, as well as raise new avenues for research. Lastly, digitally curating ancient texts in this fashion will allow smaller or marginalised fields of research, like studies of Indigenous and ancient civilisations, to join the global knowledge community and make this specialist field more present and impactful in historical research at large.

This work is licensed under Creative Commons Attribution-NonCommercial-NoDerivatives 4.0 International.