Dr Stanley Heinze, Associate Professor at Lund University’s Department of Biology, underlines his work on neuroscience, notably using elementary navigation decisions to understand brain function
This morning, I closed the front door of my house, locked it and turned around to get to work. Nothing unusual, but from the perspective of my brain there are a number of challenges to be solved within these few seconds. In principle, physical cues from the environment reach my sensory organs, my brain extracts meaningful information from these cues and transforms them into commands for my muscles.
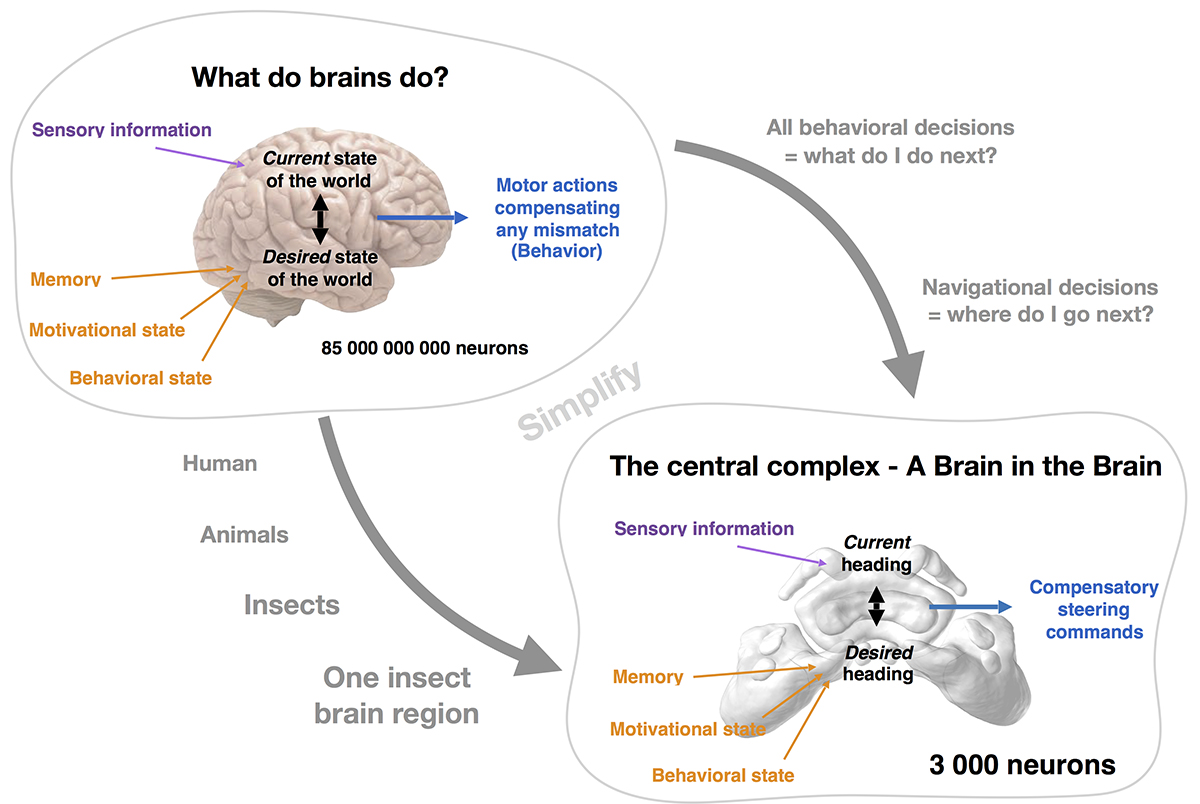
Naively, one could assume that identical sensory input would trigger identical behaviour. Yet, when facing my door, my brain instructs me to turn around and get on my bike in the morning, but to open it and go through in the evening. This is because the two views of the door are seen in different behavioural and motivational contexts (e.g. time of day, my hunger state and the knowledge of how I got into the situation).
But how do I decide what to do? In principle, the brain has to assess the current state of the world based on sensory information from the environment and compare it to an internally generated, desired state of the world.
Any mismatch between the two will trigger a behaviour to compensate for the discrepancy between what I perceive as my current world and what I would desire this world to be like. How is this desired state of the world determined by the brain? In other words, how are the goals of my actions encoded?
In humans, the answer to this question has captured the attention of philosophers and scientists since ancient times, as it ultimately relates to the concept of whether there is free will and what role conscious experiences play in how we decide upon our actions. The problem in trying to understand how our brain generates these representations, determines goals and makes decisions, is that there are 85 billion neurons in our brain, linked by more connections than there are stars in our galaxy.
there are 85 billion neurons […] more connections than there are stars in our galaxy
This results in a neural network of enormous complexity with millions of neurons involved in any of the mentioned tasks. If we would like to understand the fundamental neural processes that lie at the very heart of how brains control actions based on internal goals, this complexity has to be reduced. This is what I do in my ongoing research.
A brain in the brain
In my research group, we tackle the problem of goal-directed behaviour in the context of navigation. The ability to move from one place to another in a planned way is one of the defining features of most animals. Navigational decisions are, therefore, one of the most essential decisions that determine an animal’s chance of survival. This applies also to us humans, as most of our momentary decisions revolve around the question whether to turn right, left, or continue to go straight.
If you break down any navigation behaviour into a moment to moment basis, many can be simplified as long series of elementary navigation decisions. For these decisions, the desired state of the world simply becomes a desired movement direction. This direction has to be compared to the current body-orientation and any mismatch can be compensated by a simple steering manoeuvre. When these momentary steering decisions are chained together over time, a coherent strategy for navigation will emerge, only depending on which rules are applied to determine the desired headings. Therefore, the abstract concept of comparing the current and desired states of the world is essentially reduced to aligning two angles: the goal direction and the current heading.
Whereas this reduction in complexity is important to develop a tractable strategy to unravel the neural basis of behavioural decisions, determining navigational goals remains a highly complex task that requires many millions of neurons in the human brain and that depends on memory, internal state and highly integrated sensory information.
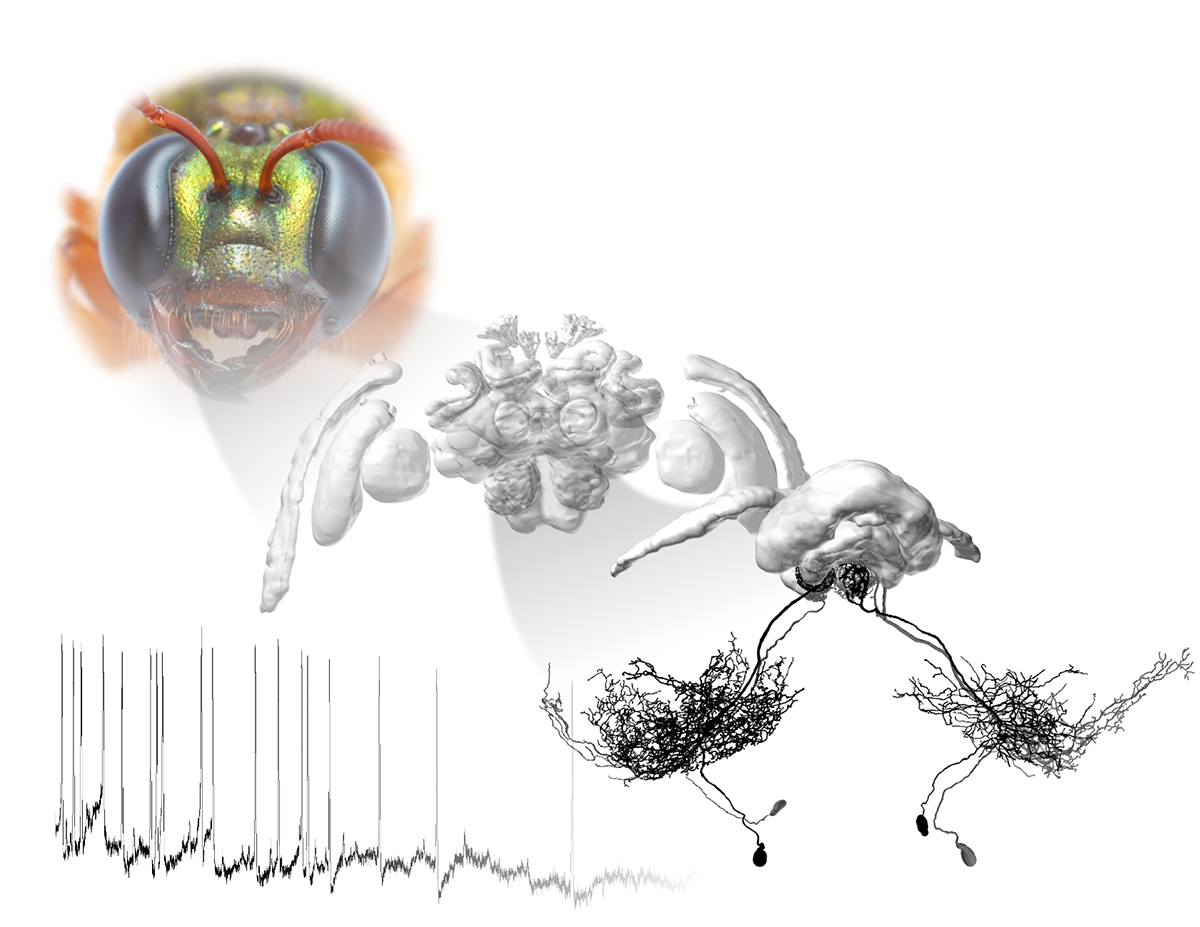
To realistically pursue the goal of understanding this process on the level of individual neurons, my research group works on insects, more specifically we focus on a single brain area in the insect brain, the central complex (CX). This region only consists of around 3,000 neurons but is involved in all of the described processes, necessary to understand brain function.
being a “brain in the brain”
It integrates multiple streams of sensory information, it is involved in visual memory, regulating internal states (e.g. sleep) and contains neurons whose activity directly controls walking behaviour. Essentially, the CX carries out all the functions that we have defined as key functions of brains in general – effectively being a “brain in the brain”.
Extracting a core circuit
The CX exists in all insects, independent of lifestyle, sensory environment and phylogenetic relationships. When comparing this region across species, its gross anatomy appears to be virtually unchanged since the dawn of insects more than 500 million years ago, suggesting that something about its function is truly essential to survival. A defining feature of the CX is its extremely regular, almost crystalline layout, consisting of 16-18 repeating vertical columns, which are intersected by horizontal layers. An interplay of two classes of neurons creates this regular array: tangential neurons transferring information from many different brain regions to this central integration hub and repeating sets of columnar neurons. The latter form stereotypical connectivity patterns within the CX and additionally constitute its main output.
The projection patterns of these neurons define the neural computations that can be carried out by the circuit. This structure-function relation is present in all brains and species but is extremely pronounced in the stereotypical projection matrix that has evolved in the CX. We found a striking example of this when plotting the tuning angles of polarized-light sensitive CX neurons against the anatomical location of their input branches. This produces a mapping of these tuning angles across the CX columns.
When the animal rotates under the sky, activity should move around this brain region in sync with the animal’s rotations. Essentially, we had found an ordered array of head-direction cells, which uses polarized skylight (and hence the Sun) as reference. Importantly, these head-direction circuits are virtually identical across all examined insects. This discovery was the first building block needed to understand the neural circuits underlying navigational decisions. But how is the information about body orientation used to drive behavioural decisions?
A model grounded in biology
An insight was offered by a chance occasion. I was waiting for a bus after a conference in Italy. At the time, I wanted to see what the patterns of connectivity in the CX were, that could be inferred from anatomical data. I had an hour and nothing better to do, so I started to draw, producing a complicated and admittedly rather messy tangle of lines, which nevertheless looked oddly familiar.
The day before, Barbara Webb, a robotics researcher from the University of Edinburgh, had given a talk about a model for path integration. Path integration is a computation that is employed by many animals, from insects to mammals and is used for finding back to a point of origin, for instance, a nest. As some insects, in particular, bees and ants, are exceptionally efficient when navigating with this strategy, I compared the individual elements of her model with my drawing, realising that every single detail could be matched to CX neurons. This was incredible – a model that had been artificially evolved by engineers to carry out path integration was embedded in the anatomical projection patterns of the bee brain!
Around the same time, I carried out electrophysiological recordings aimed at exploring how the internal compass of night-active bees differed from the Sun compass of other insects. As I could not find too many compass neurons, I used my virtual reality arena to test responses to optic flow fields that the bee would experience when flying. Indeed, I found neurons in the bee brain that responded specifically to simulated forward or backward flight. Moreover, they also projected to the CX.
Translational optic flow is used by honeybees to estimate their flight speed when using path integration. Whenever they move through their environment, the environment moves across their retina at a speed corresponding to their own speed. When this speed signal is integrated over time, the flown distance can be calculated.
During foraging, this distance is combined with compass information to keep track of every movement after the bee leaves its nest. At each point during its foraging trip, this information, therefore, tells the bee the direction and distance of the nest – its home vector. When we combined these and the remaining relevant CX neurons into a computational model, this model reliably enabled a virtual bee to find back to its nest. When implemented on a physical robot, the path integration circuit mimicking the bee CX also resulted in reliable homing behaviour.
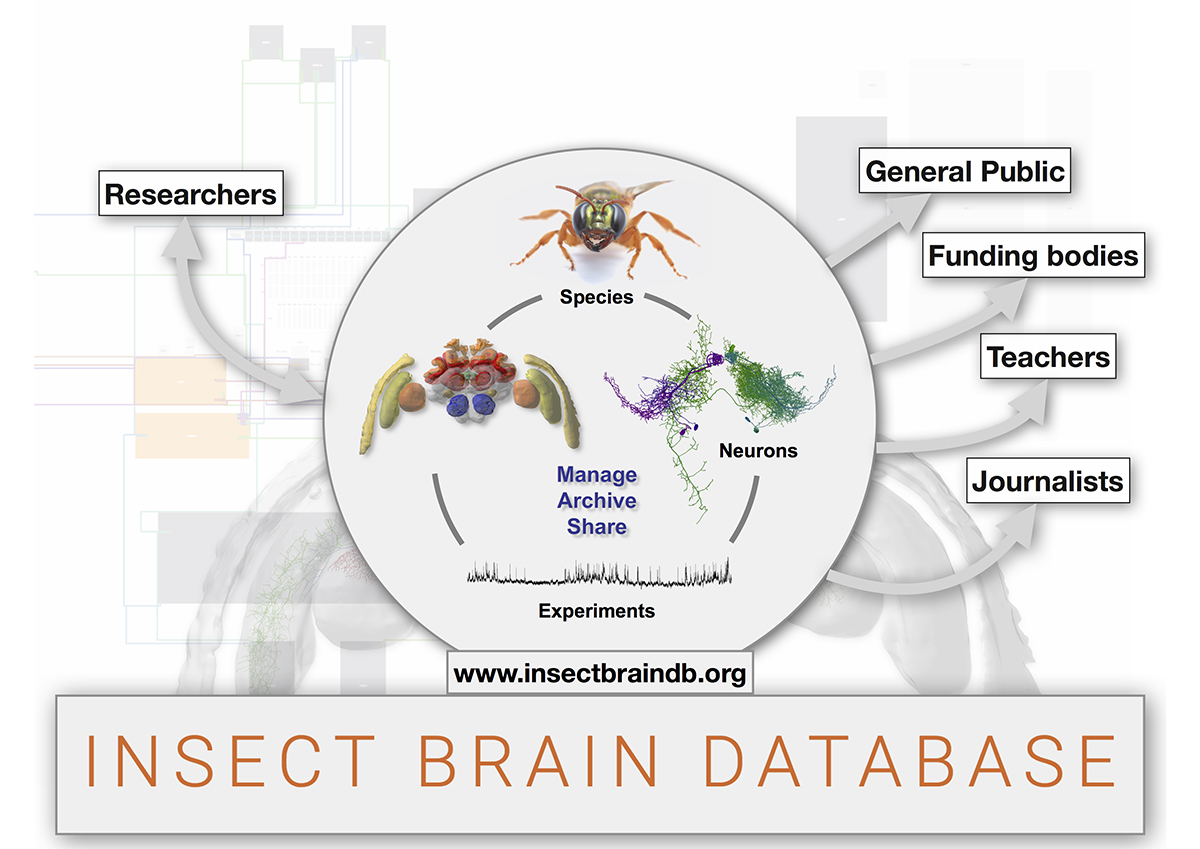
For the first time in any species, this circuit provides an anatomically constrained circuit for path integration, combining the above-described head-direction code with a similar pattern of activity that encodes the desired heading (i.e. the home vector). Both angles are represented as activity patterns, distributed across the CX columns. Steering neurons sample these two distributions and translate differences into steering commands. This circuit was what we had been looking for, a plausible neural implementation of elementary navigational decisions.
Challenges ahead
The described model circuit for path integration now provides the basis to develop a concept for how other navigation strategies could be implemented. All we needed to exchange was the source of activation in the memory neurons to generate a new desired heading, which would be compared to the animal’s current heading in the same way as during path integration, providing a basic framework to explain most directed behaviour.
While key parts of our model remain hypothetical, numerous predictions arise from the fact that all circuit functions are mapped onto existing CX neurons and, thus, can direct research to the most promising cell types. For the first time, we have an idea of what the conserved core circuit of the CX is and where the differences between species are, allowing us to observe the evolutionary tuning knobs that adapt a system to changing ecological needs.
In my group, we have started to dissect this circuit across six species of bees and ants, all differing in their mode of transportation (walking versus flying), as well as their favourite navigation strategies. Using serial section block-face electron microscopy, we are obtaining detailed connectivity maps of the CX to test circuit predictions arising from our models. A key functional prediction is that specific CX neurons encode path integration memory. This memory should depend on ongoing neural activity, similar to our own working memory (as opposed to long-term memory).
We are using molecular, anatomical, physiological and behavioural methods to detect the memory cells, confirm the nature of the memory and to develop a simple model for working memory. Excitingly, our work also begins to address the neural switches that change the mode of the path integration circuit from recording (generating the home vector) to following (using the vector to steer homewards). In essence, these questions aim at understanding the motivational inputs that decide which behaviour is appropriate in which situation.
These processes are homing in on our initial question: Why are we doing the things we do? Would it not be amazing if the basic processes underlying behavioural decisions have not dramatically changed over 500 million years and the brain of a bee could bring us closer to understanding our own human nature?
Reaching out
I have worked on insect brains for over a decade and hopefully, any reader at this point is convinced that we can gain substantial insight into how brains work from investigating these small but fascinating neural systems. Yet, if I had asked a general reader at the beginning of this essay if locust and bee brains could tell us anything about how we make decisions, the answer would have probably been a surprised: “Insects have brains?”. At least this was the response I received when I gave a presentation about my research at my old high school several years ago.
To allow insect neuroscience to reach a wider audience, I have teamed up with a professional web-developer and generated a free, online tool to visualise, manage and share original research data to further understand brain function. We are now hosting the most inclusive database on insect brain morphology and function, including data from 15 different species, based on work of ten research groups around the world. Not only are we granting access to 3D models, single neurons, images, functional information and raw data of individual experiments, but we have also developed intuitive visualisation aids that generate publication-ready illustrations for research, outreach and teaching.
To enable non-anatomists to benefit from the database, we have also generated an intuitive graphical search interface. While we are still working out the details of the long-term financial sustainability of our website it is fully functional and accessible. Using our database, scientists, journalists and members of the public can explore neuroscience data, providing an unobstructed glimpse of what the product of our research is. Ideally, this transparency will generate a deeper understanding of the output of science, might spark interest in insect neuroscience and will hopefully enhance the appreciation for the invertebrate members of the animal kingdom.
Further reading
Stone, T., Webb, B., (…) Heinze, S. (2017). An anatomically constrained model for path integration in the bee brain. Curr Biol 27, 3069–3085.e11.
Honkanen, A., Adden, A., da Silva Freitas, J. and Heinze, S. (2019). The insect central complex and the neural basis of navigational strategies. J Exp Biol 222, jeb188854.
Heinze, S. and Homberg, U. (2007). Maplike representation of celestial E-vector orientations in the brain of an insect. Science 315, 995–997.
*Please note: This is a commercial profile