Eric Frew, University of Colorado, Research and Engineering Center for Unmanned Vehicles, explores the use of unmanned aerial vehicles (UAV) for more effective cloud seeding
In Parts 1 through 3 of this four-part series we have explained how clouds form and evolve, the theory of cloud seeding, how operational seeding is implemented and why successful seeding is challenging.
Here we discuss a different approach for identifying when and where to seed a cloud using instrumented, unmanned aerial vehicles (UAV) programmed with an algorithm that implements a cloud model, coupled with measurements, to autonomously decide not only the when and where, but also the “if” seeding will be effective or detrimental.
Advantages and Shortcomings of UAVs
The primary advantage of the UAV compared to a standard aircraft is that it does not require an airport for launching and landing. This is particularly important for cloud seeding operations because oftentimes the targeted clouds might be located over regions far from the closest landing strip from which manned aircraft are launched. This means that quite often a manned seeding aircraft might get to the target regions too late for effective seeding or consume excessive fuel and flight hours ferrying back and forth or loitering while waiting for cloud formation. Likewise, manned aircraft are generally costly in terms of fuel consumption and maintenance.
The disadvantage of UAVs is that their payloads are usually limited, which means they can’t carry the same quantity of seeding material as the manned aircraft. On the other hand, being able to quickly get to the target region and make autonomous decisions for seeding means less seeding material will be required.
Example of UAV Implementation
One of the programs funded by the United Arab Emirates Rain Enhancement Program, is a collaboration between the University of Colorado (Boulder, CO), Droplet Measurement Technologies LLC (Longmont, CO) and North-West University, South Africa whereby a Super RAAVEN UAV has been instrumented with meteorological, aerosol and cloud sensors (Figure 1).
An algorithm was developed from the results of a cloud microphysical parcel model that was run several thousand times to simulate how drizzle forms as a function of updraft velocity (ww), cloud condensation nuclei (CCN) concentration, cloud base temperature and number concentration of seeding material. From these simulations, the seeding probability was parameterised such that the algorithm that runs in real time continuously measures the temperature, ww and CCN and autonomously decides whether to seed or not depending on the computed probability.
Figure 1 shows the Super RAAVEN UAV preparing to launch during a recent field operation in northeastern Colorado and the Droplet Measurement Technologies’ Cloud Droplet Probe (CDP) is shown mounted on the UAS mock-up in the laboratory. Figure 2 shows the dashboard viewed by the field scientist and illustrates how the measurements are transmitted in real time to the ground operator and displayed on the field scientist’s laptop.
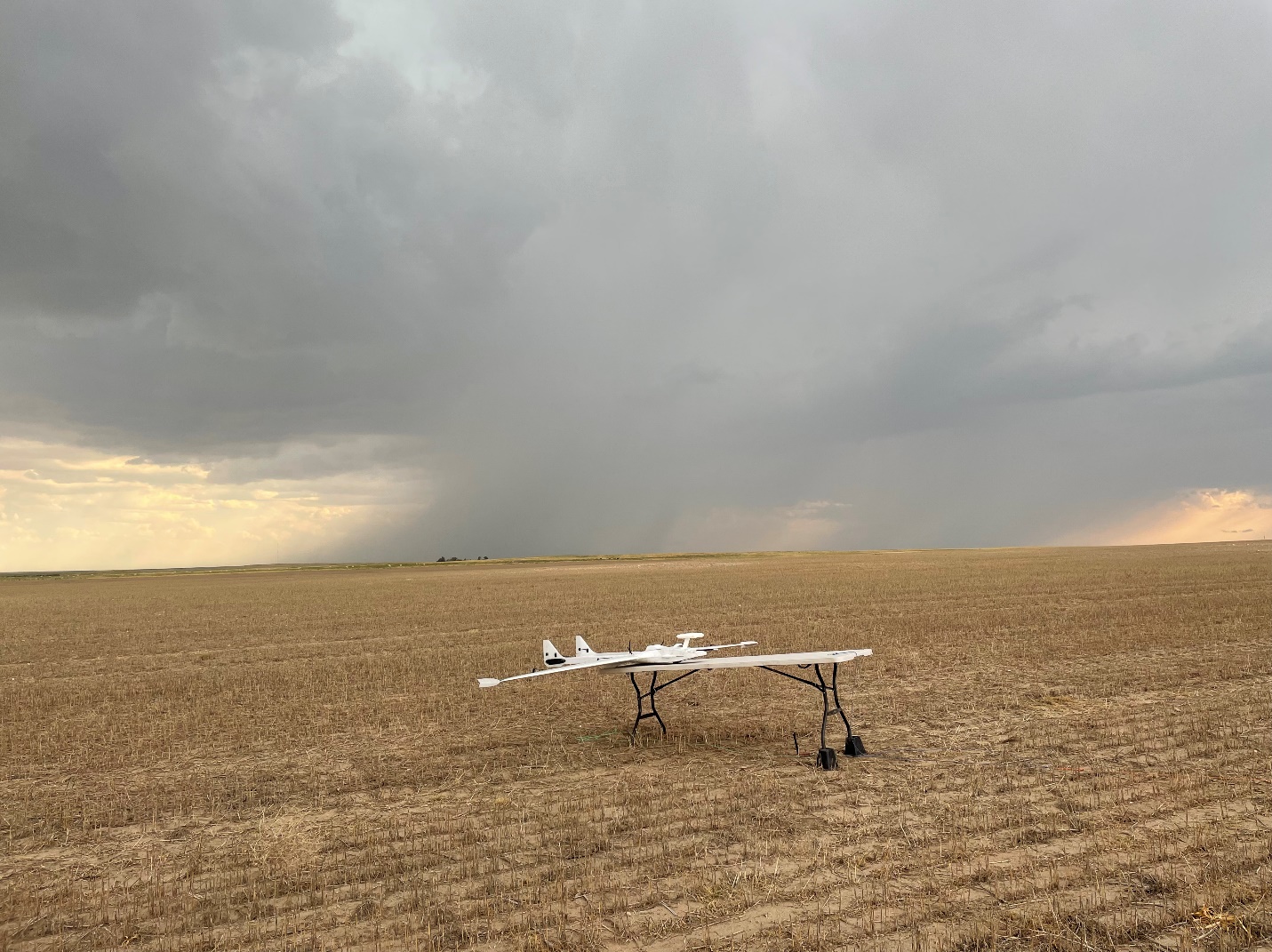
This autonomous seeding system is currently being tested in Colorado to exercise its capabilities but without actually releasing seeding material. The UAV has been successfully positioned under developing clouds and the algorithm located regions where the probability of successful seeding exceeded 50%, the threshold considered adequate to produce positive results.
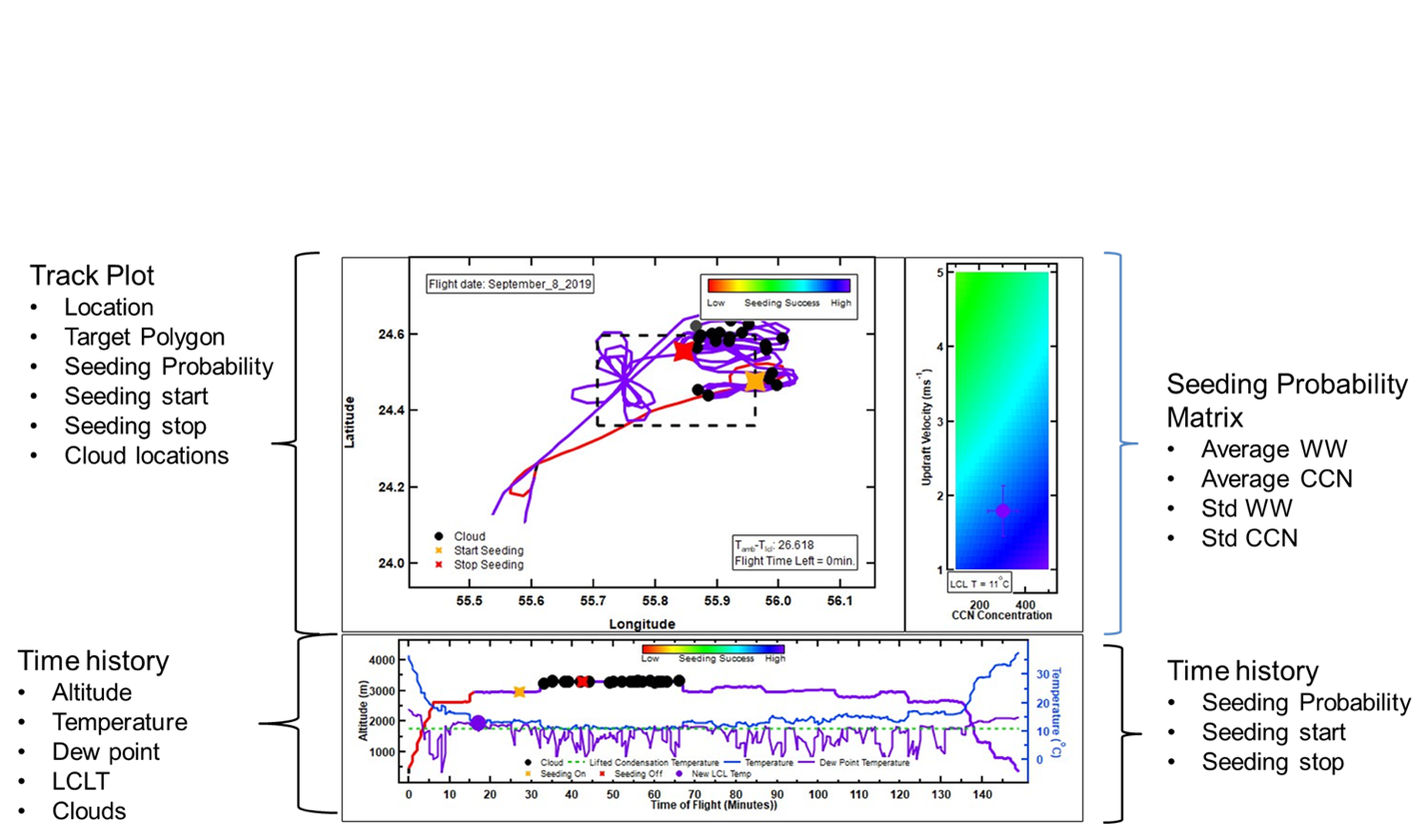
Hence, we bring to a conclusion this series of four discussions that have considered if cloud seeding can indeed mitigate climate change. While the jury is still out on the efficacy of cloud seeding in general for increasing precipitation, with new tools at their disposal, scientists will be able to better assess the optimum approach for locating those clouds where seeding can produce the desired positive effect.
Please note: This is a commercial profile